Alongside artificial intelligence, machine learning, and other similar technologies, big data is paving the way for what is called the Fourth Industrial Revolution, or ‘Industry 4.0’. With the biological, physical, and digital worlds blurring together, big data and analytics have become essential for all businesses.
As the field is constantly evolving and changing, new trends also continuously emerge. In this article, we’ll dive into big data, including:
What is big data?
Big data refers to large-scale structured, semi-structured, and unstructured datasets that are quickly created and transmitted around the world, from a wide variety of sources. With the big data analytics market having a predicted revenue of over US$68 billion by 2025, businesses need to have a developed data strategy.
As a byproduct of today’s Internet of Things (IoT), traditional tools aren’t enough for the modern types of generated data, which is constantly created when you do something as simple as opening an app. All industries contribute to this big data, including agriculture, retail, banking, tourism, and more.
Want to know more about the role of AI in banking? Read more below:
Biggest types and sources of big data
1. Publicly available data. Open data sources, such as data.gov portals from world governments.
2. Social media data. Valuable for marketing and sales, this data relates to the millions of daily interactions on platforms like Facebook and YouTube, and it includes text (comments), pictures, GIFs, voice, and more. This data is mostly unstructured or semi-structured, needing more processing before analysis.
3. Streaming data. Originating from connected devices and IoT, this data flows in chronological order into systems. It streams into IoT from smart cars, smartphones, and other devices, and can be analyzed on a continuous basis.
What are the 4 V's of big data?
Every day, there are about 2.5 quintillion bytes of data created. That's around 2.5 billion gigabytes - give or take. But how do you know if all of this data can be called ‘big’? There are typically four factors that help t determine this: variety, volume, veracity, and velocity.
1. Variety
Big data originates from a wide variety of sources, and it’s usually separated into unstructured, semi-structured, and structured data. The variety in these data types leads to the need for specialized algorithms and distinct processing capabilities. Variety makes big data big.
2. Volume
With big data, the size of data sets can often be in the terabyte and petabyte range. The volume of generated data requires processing technologies that differ from the traditional storage capabilities. The data sets in big data are too large for traditional processors, meaning companies need specialized hardware.
3. Veracity
The quality of the analyzed data is an important factor. With high-veracity data, insights can have a big impact on the overall end results, however, low-veracity data is comprised of a high volume of unimportant data. Big data acquired from various sources needs to be analyzed for context, the chain of custody, and metadata for accurate insights.
4. Velocity
Velocity is related to the speed at which new data is generated and the speed at which data moves around. With high-velocity data, such as credit card purchases, there’s a need for specialized techniques that allow for instant and real-time processing.
The extra v: value
Turning data into value is just as important. You need to have a data strategy that delivers insights and helps to make data-driven decisions, or you’ll fall behind your competitors. With effective data analysis, business operations and processes are optimized and used for the improvement of various applications.
10 top trends in big data
1. Predictive analytics
Predictive analytics help to pinpoint potential future trends from current data, thanks to statistical toolsets. By analyzing patterns in meaningful ways, predictive analytics can only offer insights and suggest the best actions to take. Industries that handle big data can then reduce business risks, boost revenue, and improve efficiency.
2. Automated artificial intelligence and machine learning
Big data analytics is ideal for powering artificial intelligence and machine learning automation, providing the needed training data for these automated tools to learn. They also allow for workflow shortcuts that help to revolutionalize business operations, as artificial intelligence combined with automation can create smart systems to automatically react to real-time situations.
Big data input for both artificial intelligence and machine learning solutions will lead to more possibilities in predictive and real-time analytics.
3. Cloud migration and storage
Helping businesses with performance, cloud migration increases speed and scalability - particularly when experiencing heavy traffic. Although it’s already been a trend for the past years, investment in cloud technology is increasing, with services like Google Cloud and Microsoft Azure offering their software as a SaaS (software-as-a-service).
Traditional data storage on-premises is also non longer enough, thanks to the terabytes and petabytes of data generated nowadays. Having either cloud or hybrid cloud solutions can simplify storage infrastructure and help with scalability.
Learn more about how hardware and software applications are helping to solve edge computing issues at the edge:
4. Data fabric
Considered a key big data trend by Gartnerin 2019, data fabric is still as relevant three years later. As an architecture and group of data services in the cloud environment, it’s comprised of data management technologies that include data governance, data pipelining, data integration, and more. Data fabric helps to:
- Support data sharing with both internal and external stakeholders through API support.
- Offer data integration and ingestion capabilities.
- Deliver built-in data preparation and data quality, which are propelled by machine learning augmented automation - leading to better data health.
5. Data regulation
When it comes to handling data on such a large scale, it’s vital to keep in mind the potential legal impacts. Certain industries like healthcare are at the core of data regulation, as it involves personal data such as mobile phone numbers, data from monitoring devices, and more.
With big data analytics, new personal data can also be created, as companies can use sensor data from cars to analyze a wide variety of factors, some of which include driving behavior. It’ll become even more important to develop strong laws to regulate big data and privacy.
Interested in the role of AI in healthcare? Then tune in to our interview series or watch on-demand.
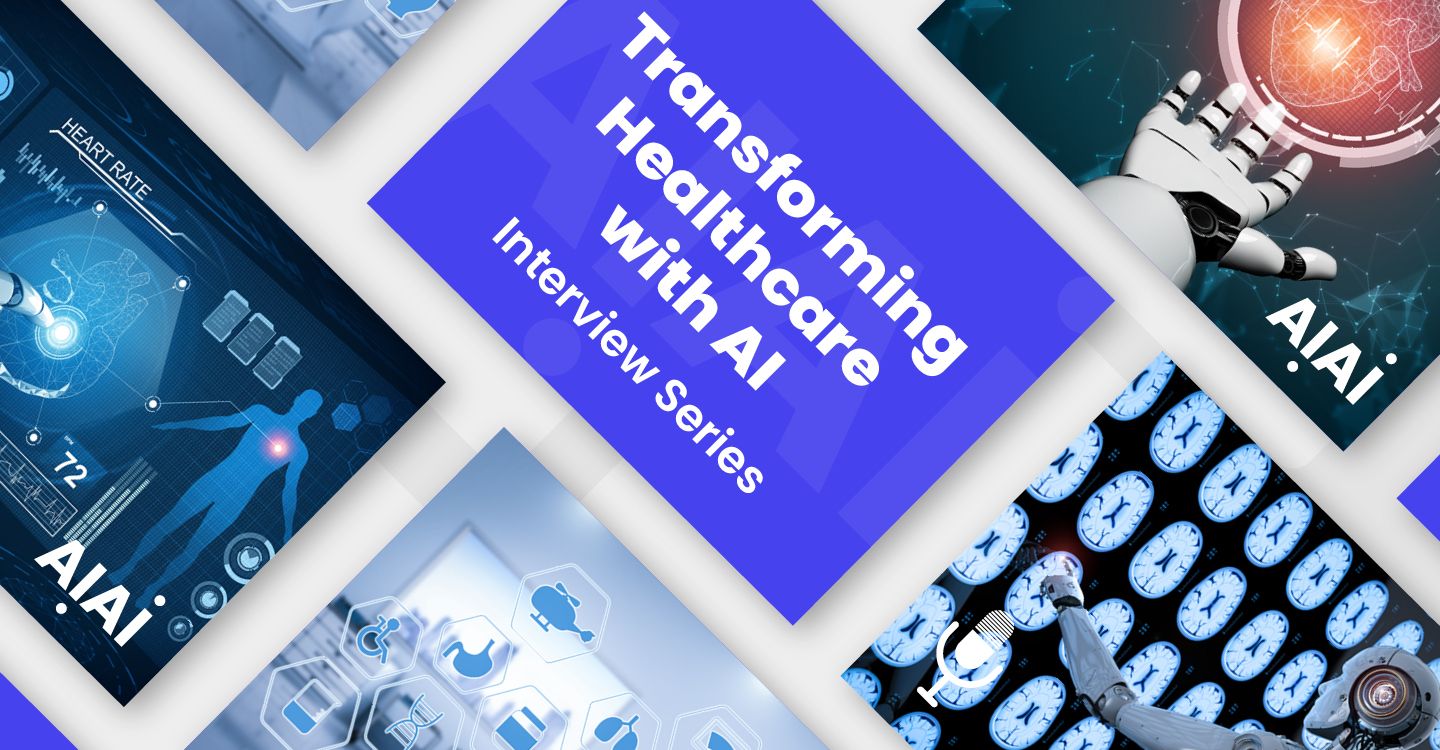
6. Data quality
Poor management of data can lead to companies acquiring data with inferior quality. Data management has to be a focus, especially when there are several mining tools involved. Data can be a big decision-maker, and it’s more and more important for businesses to have an exceptional filtration system, to prevent them from setting wrong targets due to poor quality data.
7. Blockchain for data security
Blockchain helps in effortless and secured transactions without a third-party dependency, as transactions can simply be approved by a peer network. Users can then store their encrypted data on a decentralized and secure network. Data auditing and sharing become easier, without the risk of unauthorized access. Blockchain is being utilized by e-commerce, security, and healthcare industries, for example.
8. Vector similarity search
This is a new approach to both finding and retrieving data, which can be done through deep learning. Vector similarity search helps to search through big data, indexing, and searching through vector representations of data. By utilizing a mix of algorithms and deep learning models, it finds items by conceptual meanings and not properties or keywords.
Applications can include:
- Image and audio search
- Feed ranking
- Semantic text search
- Deduplication
9. Tiny machine learning
Powered with both small and low-powered devices, like microcontrollers, tiny machine learning runs on low latency at the edge of devices. Consuming a thousand times less than a standard GPU at just milliwatts, it helps devices run for much longer - often even for years. They’re a great choice for security measures, as data doesn't get stored due to their low power consumption.
10. Cybersecurity
Remote work, for example, has come with its pros and cons, alongside the challenge of cybersecurity. As employees are outside the company's IT security range, breaches can be a big concern for some businesses. XDR, or Extended Detection Response, help in detecting cyberattacks by applying into their network advanced security analysis.
Final thoughts
In an ever-shifting digital world, advanced technology is needed for the implementation of big data trends, helping businesses reach their goals and surpass the competition. These trends are also constantly evolving and we’ve only just barely scratched the surface.
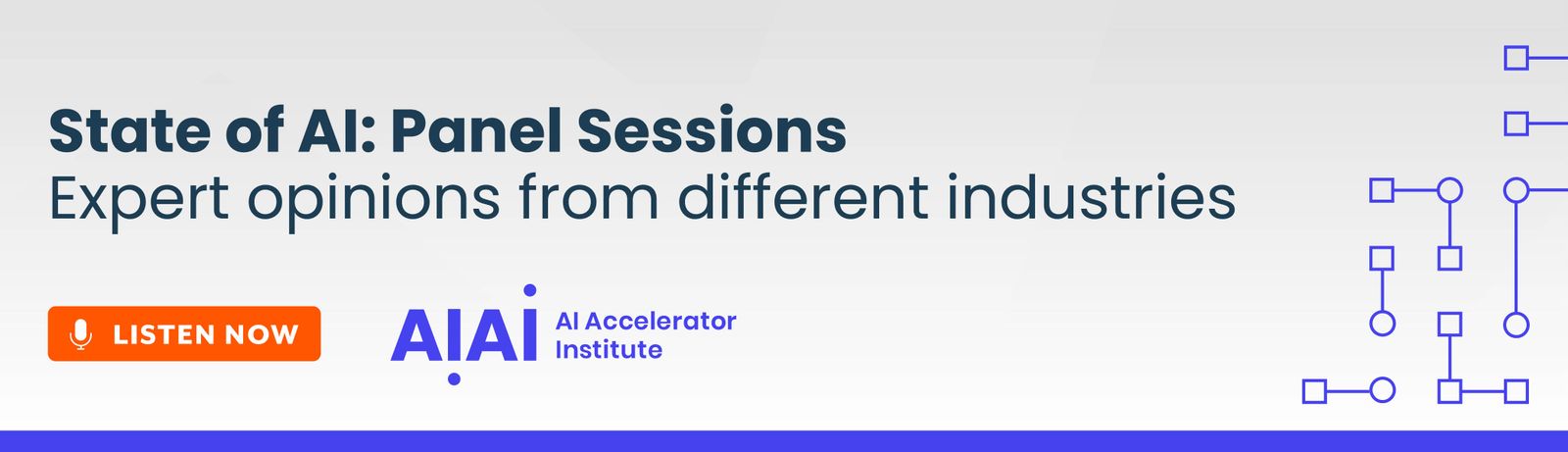