According to a report by PwC, artificial intelligence (AI) can contribute up to US$15.7 trillion to the global economy by 2030.
In the path to using the power of artificial intelligence, it can be easy to overlook the intricate challenges it can pose to sustainability. While AI is at the forefront of innovation, its impact stretches beyond technological feats, as it intertwines with environmental, ethical, and social dimensions.
In this article, we’ll go over the top 5 challenges of AI and sustainability, which are:
- Creation of e-waste
- High carbon footprint of AI models
- High resource utilization
- The problem of bias
- Lack of transparency
1. Creation of e-waste
According to the Global E-waste Monito 2020, the world will see an astonishing 74 million metric tons of e-waste by 2030. This is due to the extremely fast technological breakthroughs, the rising demand for consumer electronics, and the increasingly shorter device lifespans.
With artificial intelligence technologies being developed and integrated into daily life at a quick pace, it’s expected that the world’s e-waste problem will worsen, as there’s a higher demand for CPUs, GPUs, and memory chips.
E-waste is also leading to dangerous public health issues, with industry leaders predicting around 70% of toxic waste in landfills globally is e-waste. As it’s made of harmful substances and chemicals, it contaminates the environment around landfills.
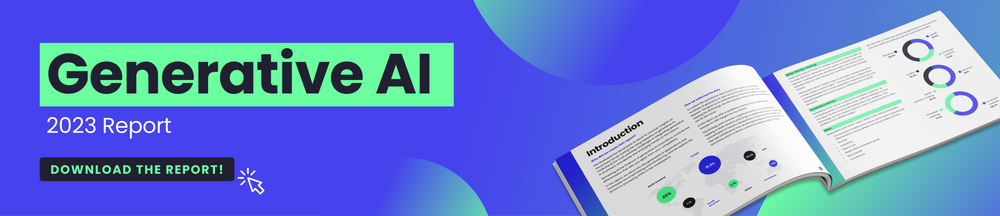
The world urgently needs a strategy to make sure the emerging e-waste crisis is addressed. Recycling electronic waste, however, is not only intricate but also labor-intensive. Taking apart electronic devices isn’t usually a simple thing to do. Manufacturers often design devices to be difficult to dismantle, aiming to prevent users from tampering with them.
Experts believe that the solution to this e-waste recycling problem could be artificial intelligence and machine learning. As these technologies allow algorithms to learn quickly and efficiently, they can achieve over 90% accuracy in identifying electronic components.
2. High carbon footprint of AI models
Google identified the “4Ms”; four best practices that, the company claims, can reduce both carbon emissions and energy by 1000x and 100x, respectively. This is available to everyone who uses the Google Cloud services. The practices are:
- Map optimization. Customers can choose the location with the cleanest energy to reduce the gross carbon footprint by 5x to 10x.
- Model. By choosing efficient machine learning models, quality can be improved while reducing computation by 3x-10x.
- Mechanization. Cloud computing reduces the amount of energy used when compared to on-premises, which leads to reduced emissions by 1.4x-2x.
- Machine. When using systems and processors that are machine learning optimized, energy efficiency and performance improve by 2x-5x.
3. High resource utilization
Data centers are known for using large amounts of water to cool their systems so the servers don’t overheat. In a recent study, researchers estimated that every ChatGPT conversation that includes 20-50 questions leads to a 500-milliliter bottle of water being “drunk” at a data center.
Google, for example, started publishing its water usage information. In 2022 alone, the company reported its data centers worldwide used 5.2 billion gallons of water. In 2023, Google planned to build a data center in Uruguay, which would consume millions of liters of water per day – something that angered the country, as they were suffering the worst drought in 74 years.
Similarly, the increase in building more data centers, energy infrastructure, and hardware has also meant an increase in the need for carbon-intensive raw materials like aluminum, silicon, plastic, copper, and more.
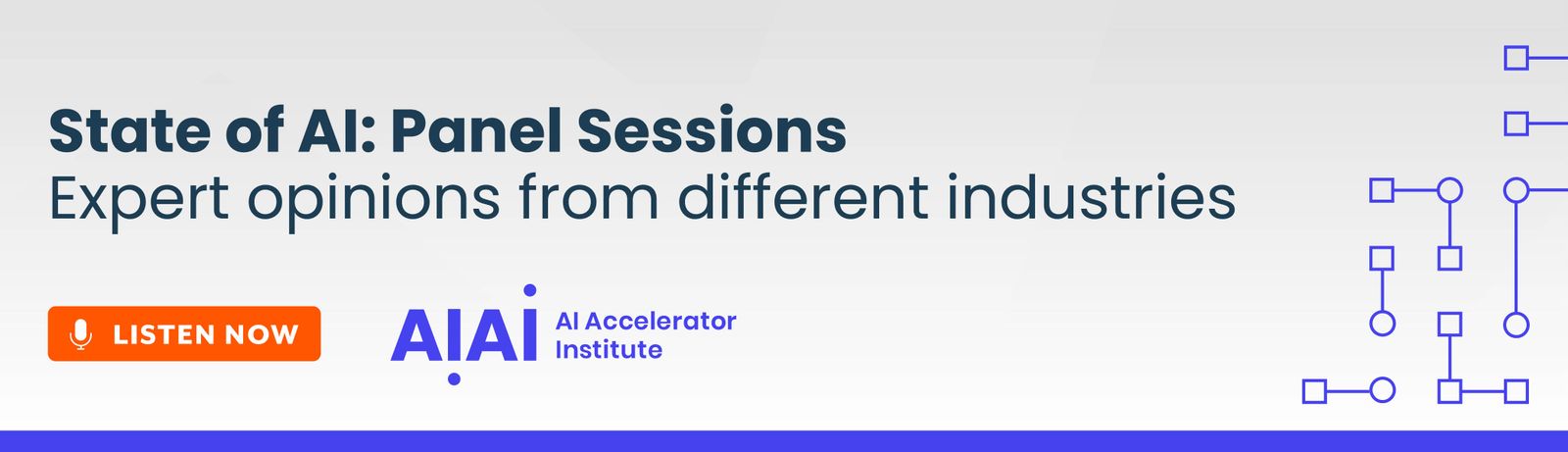
The global demand for lithium is also expected to rise to 1.1 million metric tons by 2040, which represents over 10x what’s currently being produced. All of these metals and materials need to be mined, which creates both a social and an environmental burden that’s being exported to the Global South.
Antonio del Giudice, architect and researcher, has worked with indigenous communities in Chile who’ve seen their lands used for mining lithium and copper. He says that Europe wrote off entire regions as “sacrificed zones” for mining.
4. The problem of bias
A University of Cambridge study published in npj Climate Action highlights the limitations of artificial intelligence in climate science due to biased data collection. The technology’s reliance on incomplete data, missing key perspectives, and information from various regions and communities, particularly the Global South, results in unreliable climate predictions.
The paper suggests a "human-in-the-loop" approach to fill these data gaps, making sure more accurate and inclusive AI-generated climate solutions. The study highlights the need for globally diverse data sets and the importance of broadband access as a public necessity to create socially responsible artificial intelligence tools.
“No data is clean or without prejudice, and this is particularly problematic for AI which relies entirely on digital information,” said Professor Emily Shuckburgh, co-author, Cambridge Zero Director, and climate scientist.
5. Lack of transparency
The “black box” issue in artificial intelligence refers to the lack of clarity in how machine learning models, especially deep learning networks, process data. This makes it hard to understand the decision-making process of AI, leading to concerns about accountability and bias, particularly in areas like healthcare and law.
It also restricts scalability and adoption, as users can't easily correct errors or adapt AI systems to new scenarios without understanding their inner workings. This challenge is acknowledged by many organizations, with few having comprehensive data and ethics policies.
The lack of transparency is problematic due to a variety of reasons, such as:
- Scalability. The lack of clarity in AI's decision-making process makes it challenging to identify errors or anticipate how the artificial intelligence will perform with new data. This uncertainty complicates efforts to improve or adapt AI systems for various applications, which limits their potential benefits.
- Adoption. The difficulty in understanding and explaining AI solutions is a major obstacle for organizations considering or currently implementing artificial intelligence. This lack of clarity raises concerns about decision-making risks, which stops business leaders from adopting the technologies.
- Ethical and social implications. When human operators can't grasp the logic behind AI's decisions, accountability becomes problematic, particularly in critical areas like law and healthcare. The opacity of AI systems may conceal biases and make error detection difficult.