Artificial intelligence accelerator chips, or AI accelerator chips, are being increasingly used for autonomous processes, smart devices, telecommunications, and much more. According to McKinsey & Company, it’s estimated that by 2025, AI-related semiconductors could reach $67 billion in annual sales - approximately 20% of computer chip demand.
With deep learning models getting bigger and AI-powered devices getting smaller, it becomes essential to have chips that allow AI applications to exist. From the largest providers of cloud computing to small start-ups, specialized hardware is needed to speed up AI applications like deep learning, neural networks, and machine learning.
Before you dive in, can you spare a few minutes to share your expertise in generative AI and help shape the industry?
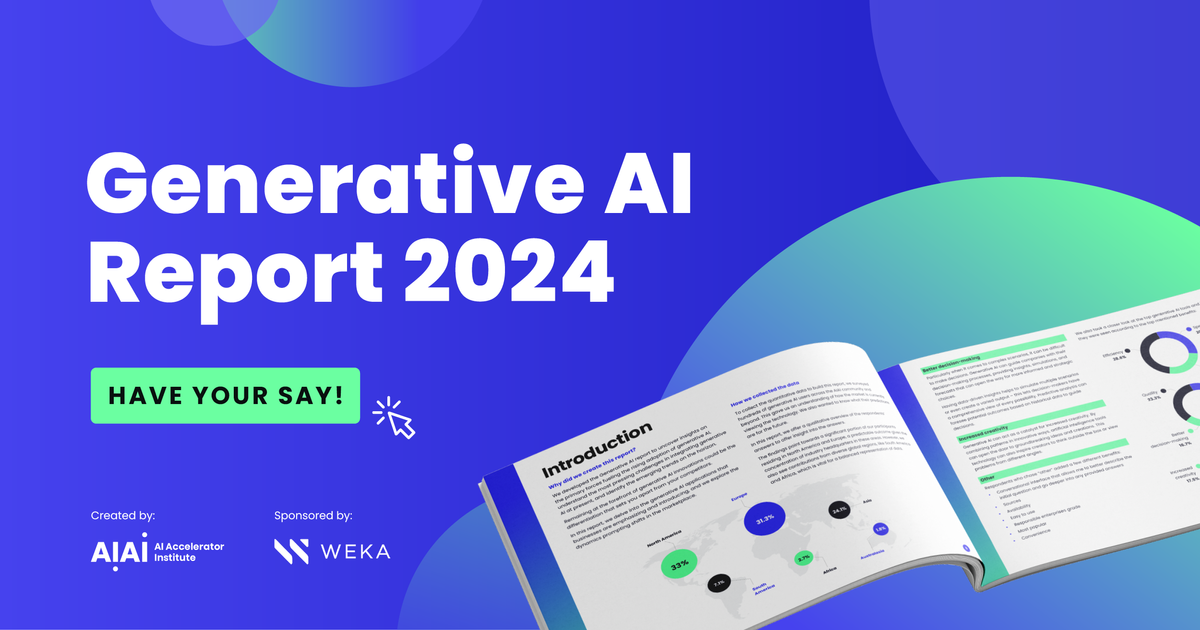
Modern AI technologies rely on a massive scale of computation, which means that training a leading AI algorithm can take up to a month of computing time and cost millions of dollars. Computer chips deliver this enormous computational power, which is specifically designed to perform unique AI system calculations efficiently.
Semiconductor chips are consistently becoming more important and their technology advancing. In 1969, the Apollo lunar module’s tens of thousands of transistors weighed 70 lb in total - today, Apple MacBooks have 16 billion transistors with a weight of 3 lb in total.
We highlighted the key chip manufacturers in our Chips of Choice 2022 report, providing an overview of these companies’ flagship chips - and why they’re great.
1. Cerebras Systems
Cerebras Systems is a team consisting of computer architects, software engineers, system engineers, and ML researchers building a new class of computer systems. Their goal is to accelerate AI and change the future of AI work.
The Chip
Source: Cerebras Systems
Wafer-Scale Engine (WSE-2)
The WSE-2 is the largest chip ever built, being 56X larger than the largest CPU. It has 123X more compute cores and 1,000X more high-performance on-chip memory. As the only wafer-scale processor ever produced, the WSE-2 has 2.6 trillion transistors, 40GB of high-performance on-wafer memory, and 850,000 AI-optimized cores accelerating AI work.
Made with the 7nm fabrication process, the chip is independently programmable and optimized for the tensor-based, sparse linear operations underpinning inference and neural network training for deep learning. Teams can train and run AI models at unprecedented scale and speed without the complex distributed programming techniques that a GPU cluster requires.
The on-wafer interconnect delivers 220 Pb/s interconnect bandwidth between cores, which represents 45,000X the bandwidth between graphic processors. This allows for a faster and more efficient execution of deep learning work at just a fraction of the power needed for traditional GPU clusters.
2. Groq
Groq focuses on key technology innovations like silicon innovation, software-defined compute, and developer velocity to deliver industry-leading performance, sub-millisecond latency, and accuracy for compute-intensive applications.
The Chip
Source: Groq
Tensor Streaming Processor
Making use of the Tensor Streaming Architecture, which is designed as a powerful single-threaded streaming processor, it has an instruction set constructed to take advantage of tensor manipulation and tensor movements for a more efficient execution of machine learning models. The interactions between memory, execution units, and other units make the architecture unique.
Equipped with a high-bandwidth inter-chip interface, it’s made to support scaling out to bigger models and to take advantage of model-level parallelism across multiple chips. It’s capable of 1 PetaOp/s performance on a single chip implementation, or one quadrillion operations per second, making it the first in the world to achieve this level of performance. It can also conduct up to 250 trillion FLOPS and has 220MB of SRAM and PCIe Gen4x16 Support.
The Tensor Streaming Processor is especially designed for the demanding performance requirements of machine learning, computer vision, and other AI-related workloads. It houses one single enormous processor with hundreds of functional units, greatly minimizing instruction-decoding overhead and handling integer and floating-point data for effortless training and best accuracy for inference.
3. Lightmatter
Born at MIT, Lightmatter is a startup with a vision of building engines with high performance, without causing a big impact on the planet. By combining photonics, electronics, and new algorithms, Lightmatter has created a next-generation computing platform that’s purpose-built for AI, without being tethered to Moore’s Law and Dennard Scaling.
The Chip
Source: Lightmatter
Envise
This general-purpose machine learning accelerator combines both transistor-based systems and photonics in one compact module. It offers offload acceleration for high-performance AI inference workloads by using a silicon photonics processing core for the majority of computational tasks.
The Envise server has 16 Envise Chips in a 4-U server configuration, consuming only 3kW power. With an unprecedented performance, it can run the largest neural networks developed to date. Each Envise Chip has 500MB of SRAM for neural network execution without leaving the processor, and 400Gbps Lightmatter interconnect fabric for large-model scale-out.
It also has an ultra-high performance out-of-order super-scalar processing architecture, 256 RISC cores per Envise processor, and a standards-based host and interconnect interface. The Envise’s specifications make it great for autonomous vehicles, predictive and preventative maintenance, cancer detection, text-to-speech and language translation, vision and control in robotics, and much more.
4. SambaNova Systems
Founded in 2017, the American company SambaNova Systems is creating the next generation of computing to bring AI innovations to organizations across the globe. The SambaNova Systems Reconfigurable Dataflow Architecture powers the SambaNova Systems DataScale, from algorithms to silicon - innovations that aim to accelerate AI.
The Chip
Source: SambaNova Systems
Cardinal SN10 RDU
The 40 billion transistor reconfigurable dataflow unit, or RDU, is built on TSMC’s N7 process and has an array of reconfigurable nodes for switching, data, and storage. The chip is designed for in-the-loop training and model reclassification and optimization on the fly during inference-with-training workloads.
Each chip has six controllers for memory, which allows for 153GB/s bandwidth. Sold as a solution to be installed in data centers, the Cardinal SN10 RDU is a next-generation processor for programmable acceleration and native dataflow processing.
The basic unit, the DataScale SN10-8R, features an AMD processor that is paired with eight Cardinal SN10 chips and 12 terabytes of DDR4 memory - the equivalent of 1.5TB per Cardinal.
The Cardinal SN10 has a tiled architecture consisting of a network of reconfigurable functional units, and the architecture allows for a wide range of highly parallelizable patterns within the dataflow graphs to be programmed as a mix of memory, compute, and communication networks.
5. Tenstorrent
Toronto-based Tenstorrent is a machine learning and artificial intelligence startup company specializing in AI structures focused on scalable adaptability and deep learning. The company was founded by engineers and leaders from semiconductor companies and has taken an approach to end unnecessary computation to break the direct link between compute/memory bandwidth and model size growth requirements.
Their architecture is composed of a Tensix core array, which is proprietary, each having a powerful, programmable SIMD and dense math computational block alongside five flexible and efficient single-issue RISC cores.
The Chip
Fast Grayskull. Source: Tenstorrent
Grayskull
Enabling conditional execution, which allows for faster AI inference and training and workload scaling support from edge devices to data centers, Grayskull has 120 Tenstorrent proprietary Tensix cores. Each of these cores has a high-utilization packet processor, a dense math computational block, a programmable single-instruction multiple data processor, and five reduced-instruction set computer cores.
The Tensix array is paired with 120MB of local SRAM and eight channels of LPDDR4 that support as much as 16GB of external DRAM and 16 lanes of PCIe Gen4. Stitched together with a double 2D torus network-on-chip, which makes multicast flexibility easier, the Tensix array has minimal software burden for scheduling coarse-grain data transfers.
The chip can achieve 368 TOPS and as much as 23,345 sentence/second at the chip thermal design power set-point needed for a 75W bus-powered PCIe card, using BERT-based for the SQuAD 1.1 data set. Grayskull is ideal for public cloud servers, inference in data centers, automotive, and on-premises servers.
6. Mythic
Mythic is a company of leading experts in neural networks, software design, processor architecture, and more, all focused on accelerating AI. They’ve developed a unified software and hardware platform with a unique Mythic Analog Compute Engine, the Mythic ACE™, that delivers power, performance, and cost to enable AI innovation at the edge.
The Chip
Source: Mythic
Mythic MP10304 Quad-AMP PCIe Card
Enabling high performance for power-efficient AI inference in both edge devices and servers, the PCIe card simplifies integration effort into platforms where there is a constraint of space. With four M1076 Mythic Analog Matrix Processors, or AMPs, it delivers up to 100 TOPSf AI performance and supports up to 300 million weights for complex AI workloads below 25W of power.
The PCIe card can also have large DNN models deployed by using the combined AI compute of the four M1076 Mythic AMPs. It also runs smaller DNN models for video analytics applications that process images from a variety of cameras. It features on-chip storage of model parameters, 4-lane PCIe 3.0 for up to 3.9GB/s bandwidth, OS support, and more.
It allows for complex AI networks to be deployed in network video recorders, or NVRs, and edge appliances to capture video data from multiple cameras in the field. It can also deploy complex networks at a high resolution for applications that need high accuracy.
7. Sima.ai
Enabling high-performance compute at the lowest power, Sima.ai is a machine learning company. Led by a team of technology experts who are committed to delivering the highest frames-per-second-per-Watt in the industry, Sima.ai’s initial focus was on delivering solutions for computer vision applications.
The Chip - MLSoC™
Designed with low power needs and support for fast inferencing, Sima.ai’s AI accelerator chip has performance ranges between 50-200 TOPS, or theoretical operations per second, at 5-20 Watts - an industry first at 10 TOPS per Watt, according to Sima.ai.
Combining traditional compute IP from Arm, the Arm Cortex-A65 CPU, with the company’s own machine learning accelerator and dedicated vision accelerator, and combining multiple machine learning accelerator mosaics through a proprietary interconnect, the chipset can theoretically be scaled up to 400 TOPS at 40 Watts.
It’s originally designed for computer vision applications, but it’s capable of a range of machine learning workloads like natural language processing.
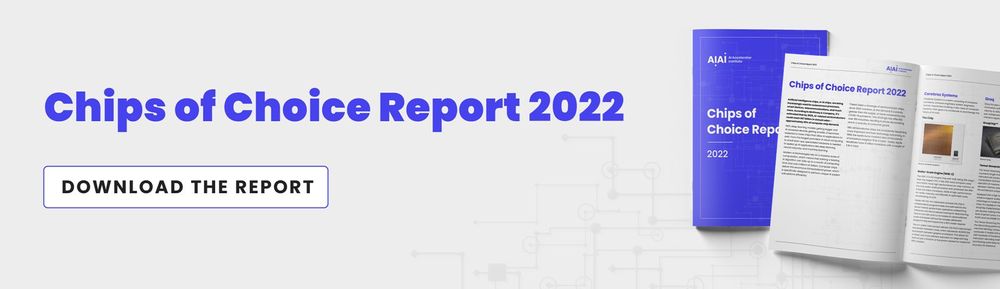
8. NVIDIA
NVIDIA is an American multinational technology company that designs GPUs for professional and gaming markets, alongside System-on-Chip units for the automotive market and mobile computing. They invented the GPU in 1999, which propelled the growth of the PC gaming market and redefined modern computer graphics, artificial intelligence, and high-performance computing.
The company works on AI and accelerated computing to reshape industries, like manufacturing and healthcare, and help grow others. NVIDIA’s professional line of GPUs is used throughout several fields, such as engineering, scientific research, architecture, and more.
They also offer CUDA, an application programming interface, or API, that allows for the creation of massively parallel programs that use GPUs, which are deployed in supercomputing sites across the globe. NVIDIA recently announced plans to acquire Arm Ltd., a semiconductor and software design company.
NVIDIA AI chips, and quantum computing services, are helping to develop general-purpose humanoid robotics.
The Chip
Source: NVIDIA
Grace
The company’s first data center CPU, Grace, offers 10X performance for systems that train giant AI models by using energy-efficient Arm cores. Designed for the computing requirements of the world’s most advanced applications, like AI supercomputing and natural language processing, it helps to analyze huge datasets that need massive memory and ultra-fast compute.
Grace is supported by the NVIDIA HPC software development kit and the full suite of CUDA® and CUDA-X™ libraries. These accelerate over 2,000 GPU applications. At the center of the chip’s performance is the fourth-generation NVIDIA NVLink® interconnect technology, which offers a record 900GB/s connection between the chip and NVIDIA GPUs. This allies for 30X higher aggregate bandwidth against modern leading servers.
It also has an innovative LPDDR5x memory subsystem for twice the bandwidth and 10X better energy efficiency in comparison to the DDR4 memory. The new architecture also offers a unified cache with a single memory address space, GBM GPU memory for simplified programmability, and a combining system.
9. Intel
American multinational corporation and technology company Intel is one of the world’s largest semiconductor chip manufacturers. They developed the x86 series of microprocessors, which are found in most PCs.
Intel supplies its microprocessors to computer system manufacturers like HP and Lenovo, while also manufacturing graphics chips, motherboard chipsets, integrated circuits, embedded processors, and more.
The Chip
Source: Intel
11th Gen Intel® Core™ S-Series
Designed for faster and easier work, the 11th Gen Intel® Core™ has AI-assisted acceleration, best-in-class wireless and wired connectivity, and Intel® Xe graphics for improved performance. Redefining the company’s CPU performance for both desktop and laptop, it has new core and graphics architectures.
The 11th Gen Intel® Core™ processors built on the Intel vPro® platform offer modern remote manageability and hardware-based security to IT, making it ideal for business. The S-Series desktop processors offer improved performance by taking advantage of Intel® Deep Learning Boost to accelerate AI performance.
The S-Series has up to 20 CPU PCIe 4.0 lanes, memory support up to DDR4-3200, Intel® Optane™ memory H20 with SSD support, and integrated USB 3.2 Gen 2x2 (20G). All of these technologies combine power and intelligence that supercharge productivity.
Intel® Deep Learning Boost (VNNI) accelerates AI inference, which greatly improves performance for deep learning workloads. The Gaussian Neural Accelerator 2.0 (GNA 2.0) runs AI workloads on an accelerator so that background noise is better suppressed and video background more efficiently blurred.
11th Gen Intel® Core™ desktop processors are ideal for creators and gamers, improving productivity, graphic design, and video editing.
10. Graphcore
The British semiconductor company, Graphcore, develops accelerators for machine learning and AI, aiming to make a massively parallel intelligence processing unit, or IPU, holding the complete machine learning model inside the processor.
The company believes its IPU technology can become the standard across the globe for machine intelligence compute. As it’s a completely new processor, the IPU has been designed for AI compute, letting researchers conduct advanced work in fields like decarbonization and drug discovery.
The Chip
Source: Graphcore
Colossus™ MK2 GC200 IPU
The 2nd generation Colossus™ MK2 GC200 IPU processor is a new massively parallel processor to accelerate machine intelligence, which was co-designed from the ground up with Poplar® SDK.
The Poplar® SDK is a complete software stack that helps implement Graphcore’s toolchain in a flexible and easy-to-use software development environment. It seamlessly integrates with TensorFlow 1 and 2 (with full performant integration with TensorFlow XLA backend), PyTorch for targeting IPU by using PyTorch ATEN backend, PopART™ (Poplar Advanced Runtime) for training and inference, supporting Python/C++ model building plus ONNX model input, and more.
The Colossus™ MK2 GC200 has 59.4 billion transistors and it was built with TSMC’s 7N process. With 1,472 powerful processor cores that run almost 9,000 independent parallel program threads, it has an unprecedented 900MB In-Processor-Memory™ with 250 teraFLOPS of AI compute at FP16.16 and FP16.SR, or stochastic rounding.
The 2nd generation Colossus has allowed Graphcore to develop groundbreaking advances in communication, compute, and memory in their silicon and systems architecture. It achieves an 8X step up in real-world performance in comparison to Graphcore’s MK1 IPU.
11. Arm
UK-based semiconductor and design software company Arm is owned by SoftBank. Although Arm doesn’t manufacture the semiconductors itself, it licenses its own designs. The company looks to offer machine learning capabilities designed for power-efficient sensors, low-cost, and electronics.
Mainly dealing with the design of ARM processors (CPUs), it also designs systems and platforms, software development tools under Keil, DS-5, and RealView brands, System-on-Chip infrastructure, and software.
The Chip - Cortex-M55
Bringing endpoint AI to billions, the Cortex-M55 is the company’s most AI-capable Cortex-M processor. It’s also the first one to feature Arm Helium vector processing technology for energy-efficient and enhanced digital signal processing, or DSP, and machine learning performance.
Offering an easy way of implementing AI for the Internet of Things with the ease of use of Cortex-M, an industry-leading embedded ecosystem, optimized software libraries, and a single toolchain.
It offers up to 15X machine learning performance improvement and up to 5X signal processing performance uplift in comparison to existing Cortex-M processors. The Cortex-M55 can be integrated with Cornerstone-300, which includes a pre-verified subsystem and system IP that helps System-on-Chip designers to more quickly build secure systems.
The Ethos-U55 neural processing unit is designed to run with the Cortex-M55, offering up to 480X increase in AI performance in both energy-constrained devices and area with a single toolchain.
12. Qualcomm
American multinational corporation Qualcomm creates semiconductors, wireless technology services, and software solutions. Mainly a fabless manufacturer, it also develops software and semiconductor components for laptops, vehicles, watches, smartphones, and more.
The company focuses on breakthrough technologies that allow for the transformation of how the world computes, connects, and communicates. 5G-enabled smartphones and other products and technologies are possible due to Qualcomm’s developments.
The Chip
Source: Qualcomm
Cloud AI 100
Designed for AI inference acceleration, the Cloud AI 100 addresses specific requirements in the cloud, such as process node advancements, power efficiency, signal processing, and scale. It eases the ability of data centers to run inference on the edge cloud much faster and more efficiently.
It was built with the 7nm process node and has 16 Qualcomm AI cores, which achieve up to 400 TOPs of INT8 inference MAC throughput. The memory subsystem has four 64-bit LPDDR4X memory controllers that run at 2100MHz. Each of these controllers runs four 16-bit channels, which can amount to a total system bandwidth of 134GB/s.
Balancing out what may seem like a slim bandwidth, Qualcomm uses a massive 144MB of on-chip SRAM cache to make sure it keeps as much memory traffic as possible on-chip. Larger kernels will require workloads to be scaled out over several Cloud AI 100 accelerators.
The chip’s architecture supports INT8, INT16, FP16, and FP32 precisions, giving it flexibility in terms of models that are supported. There’s also a set of SDKs to support a set of industry-standard frameworks, exchange formats, and runtimes.
13. Flex Logix
American technology company Flex Logix provides industry-leading solutions that enable flexible chips and accelerate neural network inference. It develops and licenses software solutions and programmable semiconductor IP cores.
Flex Logix claims that its technology offers cheaper, faster, and smaller FPGAs that use less power.
The Chip
Source: Flex Logix
InferX X1
Delivering more performance at a lower price, the chip has low latency and very high accuracy. It’s supported by a very small die size of 54 mm2 in TSMC’s 16FFC, with a total design power of 7-13W. It has Tensor processors that do the inference work, and PCIe boards for applications with a form factor that needs additional hardware.
The compiler for the chip is extremely simple to use, reading in a high-level representation of the algorithm in either ONNX or TensorFlow Lite. The compiler then makes decisions about the best way of configuring the chip for the target application.
With its unique TPU array architecture, the chip has a focus on challenging edge vision applications by delivering leading-edge performance while still being flexible so that customers can seamlessly integrate into new AI models in the future.
14. AMD
AMD is an American multinational semiconductor company that develops powerful computer processors and power devices. Some of their products include embedded processors, microprocessors, graphics processors for servers, motherboard chipsets, embedded system applications, and more.
The Chip
Source: AMD
3rd Gen AMD EPYC™ 7003 Series
The EPYC™ 7003 series processors offer outstanding performance for a wide range of industry-standard applications. Built on both AMD Infinity Architecture and “Zen3” core, the processors have a full feature set across the stack with an integrated security processor on-die, industry-leading I/O, and 7 nanometer x86 CPU.
They also offer up to 32M of L3 cache per core, performance in multiple DIMM configurations, 4-6-8 channel interleaving for more configuration flexibility, and synchronized clocks between fabric and memory. Specially engineered for data centers relying on throughput and GPU performance, the processors scale from 8 to 64 cores, or 16 to 128 threads per socket.
15. TSMC
TSMC, or Taiwan Semiconductor Manufacturing Company, has a focus on manufacturing products for customers. Their semiconductors serve a global customer base with a wide range of applications, from mobile devices to automotive electronics and more.
With the announcement of an advanced semiconductor fab to be built and operated in the US by 2024, TSMC’s Arizona-based facility will use its 5-nanometer technology to fabricate up to 20,000 semiconductor wafers per month.
The chip technology
N7 technology. Source: TSMC
7nm
Setting the industry standard for 7nm process technology development, TSMC’s 7nm Fin Field-Effect Transistor, or FinFET N7, delivers 256MB SRAM with double-digit yields. Compared to the 1-nm FinFET process, the 7nm FinFet process has 1.6X logic density, ~40% power reduction, and ~20% speed improvement.
Launching two separate 7nm FinFET tracks, TSMC set another industry record; a track optimized for mobile applications and another track for high-performance computing applications.
For 2022, TSMC plants to launch both 4nm (N4) and 3nm (N3) technologies. The N4 will be an enhanced version of the N5, offering more enhanced performance, density, and power for the next batch of N5 products. The N3 will deliver the most advanced foundry technology for both transistor technology and PPA. It will also offer up to 70% power reduction at the same speed, in comparison to the N5.
16. Apple
The world’s 4th largest PC and smartphone vendor, Apple is an American multinational company that designs, manufactures, and markets PCs, smartphones, tablets, wearables, and more. Since 2020, Apple has announced the transition of its Mac personal computers from Intel processors to in-house developed processors.
The MacBook Air, MacBook Mini, and MacBook Pro were the first Mac devices to be powered by Apple-designed processors - the Apple M1.
The Chip - A15 Bionic
Announced for the iPad mini 6, iPhone 13 Pro, and iPhone 13 Pro Max models, the A15 Bionic is a System-on-Chip with 15 billion transistors (that’s a jump from the 11.8 billion on the A14 in the iPhone 12 models), new graphics, machine learning tasks, and AI capabilities.
Featuring two high-performance cores (Avalanche) and four energy-efficiency cores (Blizzard), it was built on TSMC’s 5-nanometer manufacturing process. It has a 16-core neural engine dedicated to speeding up all artificial intelligence tasks and capable of performing 15.8 trillion operations per second, which is a rise from the A14’s 11 trillion operations per second.
Its new image signal processor has improved computational photography abilities, and the system cache boasts 32MB. The A15 also has a new video encoder, a new video decoder, a new display engine, and wider lossy compression support.
17. Xilinx
Delivering dynamic processing technology and enabling rapid innovation with adaptable and intelligent computing, Xilinx invents programmable System-on-Chips, FPGA, and ACAP. Their highly-flexible programmable silicon is enabled by a suite of advanced software and tools, driving rapid innovation for both industries and technologies.
Xilinx builds user-friendly development tools, accelerates critical data center applications, and grows the compute ecosystem for machine learning, video and image processing, data analytics, and genomics.
The Chip
Source: Xilinx
Versal® AI Core Series
Delivering the highest compute and lowest latency in the Versal products, the Versal AI Core series allows for breakthrough AI inference performance and throughput in its AI engines. It’s been optimized for compute-intensive applications mainly for A&D markets, data centers, and 5G wireless. This also includes advanced signal processing and machine learning.
Combining PCIe® Gen4/Gen5 compliance, multi-rate Ethernet MACs supporting several ethernet configurations that maximize flexibility and connectivity, CCIX support, and high-performance GPIO, it also features rearchitected low latency of 32.75Gb/s transceivers.
The AI Engines offer up to 5X higher compute density for vector-based algorithms, and it’s also optimized for AI/ML computation and real-time DSP. The enhanced DSP engines offer support for single and half-precision floating-point and complex 18x18 operations. The power-optimized VDUs, or video decoder units, have multiple VDEs, or video decoder engines. VDUs are excellent for image processing applications in which various video inputs feed a central hub that both decodes and executes advanced machine learning algorithms.
The Versal® AI Core Series is ideal for data center compute, wireless test equipment, medical image processing, 5G radio and beamforming, radar processing, and video processing for smart cities.
18. MediaTek
As the 4th largest global fabless semiconductor company, MediaTek builds chips for smartphones, smart televisions, voice assistant devices, Android tablets, feature phones, and optical disc products.
Their chipsets share core technologies across Mobile, Home, and Automotive platforms to ensure all segments of markets can benefit from a single piece of their intellectual property.
The chipsets are also optimized to run cool and extremely power-efficient so that battery life is extended.
The Chip - Pentonic 2000
MediaTek’s new flagship System-on-Chip, the Pentonic 2000, was created for flagship 8K televisions with up to 120Hz refresh rates. Announced to launch in 2022 as the “fastest” GPU and CPU on this market, it’s the first smart-screen System-on-chip based built with TSMC’s advanced N7 nanometer process. It also has an ultra-wide memory bus and ultra-fast UFS 3.1 storage, alongside support for fast wireless connectivity for MediaTek Wi-Fi 6E or 5G cellular modems.
With a range of cutting-edge technologies, it has 8K MEMC and AI engines and can provide astonishing cinematic experiences in Dolby Vision and Dolby Atmos. With MediaTek’s AI processing engine (APU) fully integrated into the Pentonic 2000, processing engines are faster and more power-efficient than multi-chip solutions.
The Pentonic 2000 has AI-enhanced features such as AI-Voice technologies, AI-Super Resolution 8K, 2nd Generation AI-Picture Quality Scene Recognition, and 3rd Generation AI-Picture Quality Object Recognition.
19. IBM
IBM, or International Business Machines Corporation, is an American multinational technology company that produces and sells computer software, hardware, and middleware. They also offer consulting services and hosting in areas such as nanotechnology.
Additionally, they also conduct research and hold patents for inventions such as the hard disk drive, the SQL programming language, the magnetic stripe card, and more. Both computers and employees from IBM helped NASA track orbital flights of the Mercury astronauts in 1963, and the company went on to support NASA with space exploration for the rest of the 1960s.
Presently, IBM has two separate public companies, with IBM’s focus for the future on high-margin cloud computing and artificial intelligence.
The Chip
Credit: Connie Zhou for IBM. Source: IBM
Telum
Planned for the first half of 2022, Telum has been designed for low latency, real-time AI to allow it to be embedded in transaction workloads. It has eight processor cores, which run at more than five gigahertz, executing the program. Each of the eight cores is connected to a 32MB private L2 cache, containing the data allowing programs to access the data to operate at high speeds.
All the caches are then connected with a ring that sends data between them when the different programs are communicating with each other. The result - 256MB of cache. The Telum chip is a combination of AI-dedicated functions and server processors capable of running enterprise workloads.
20. GrAI Matter Labs
Dealing with life-ready AI, GrAI Matter Labs’ goal is to create artificial intelligence that feels alive and behaves like humans. These brain-inspired chips help machines make decisions in real-time, optimize energy, save money, and maximize efficiency.
The Chip
Source: GrAI Matter Labs
GrAI VIP
The GrAI VIP (Visual Inference Processor) is a full-stack AI System-On-Chip delivering Resnet-50 inferences at ~1ms. It has the fastest AI processing at low power due to leveraging time-sparsity to enable inference times in milliseconds. It’s got system latency reduction with interfaces allowing decisions to be made at the sensor. And, it also has support for standard camera sensors without needing event-based data sets.
The GrAI VIP (Vision Inference Processor) is the first GrAI Matter Labs product chip that has been awarded the CES-2022 Innovation Honoree. This chip will allow customers and partners to build Life-Ready AI products.
FAQs
What is an AI chip?
An AI chip is a type of specialized hardware designed to efficiently process AI algorithms, especially those involving neural networks and machine learning.
Why are AI chips important?
AI chips are crucial for accelerating AI applications, reducing computational times, and improving energy efficiency, which can be pivotal in applications like autonomous vehicles, smart devices, and data centers.
What factors should I consider when choosing an AI chip?
Key factors include computational power, energy efficiency, cost, compatibility with existing hardware and software, scalability, and the specific AI tasks it is optimized for, such as inference or training.
What’s the difference between training and inference in AI chips?
Training AI chips are designed for building and training AI models, which requires significant computational power and memory. Inference chips, on the other hand, are optimized for executing these models to make decisions based on new data.
How do I compare different AI chips?
Look at benchmarks such as performance on specific tasks, power consumption, processing speed, support for software libraries and frameworks, and real-world application performance reviews.
Are there any industry standards I should be aware of when choosing an AI chip?
While there are no universal standards, aligning with popular machine learning frameworks like TensorFlow or PyTorch can be beneficial. Additionally, compatibility with industry-standard interfaces and protocols is important.
Can AI chips be used in consumer devices?
Yes, AI chips are increasingly found in consumer devices like smartphones, tablets, and home automation systems to improve functionalities like voice recognition, image processing, and user interaction.
What are the challenges in using AI chips?
Challenges can include high costs, complexity of integration into existing systems, rapid obsolescence due to fast-paced technology advances, and the need for specialized knowledge to develop and deploy AI applications.