Data is an extraordinary tool that can help marketers better understand their customers and predict their behavior. Armed with all this data, marketers can specifically tailor their direct marketing efforts to the right kinds of people, significantly increasing their chances of a sale.
Direct marketing refers to how we directly communicate with target audiences, delivering messages via direct channels like social media, phone calls, and email campaigns. So much data can be accrued from this kind of marketing, and machine learning is a crucial tool for managing this substantial data.
Machine learning is a subset of AI that uses data to answer questions. One of the key benefits of machine learning is its ability to “learn” so it can make predictions or decisions without being specifically programmed to do so.
56.5% of marketers are using machine learning for content personalization. It can be used to learn the patterns of website users and predict their future behavior. Using these behavioral and demographic clues, it can devise individual predictions for how likely a consumer is to buy at that moment.
In this article, we’re going to take a closer look at machine learning and how it can be applied to direct marketing, as well as some real-world examples of how machine learning is already being used.
Read more about machine learning below:
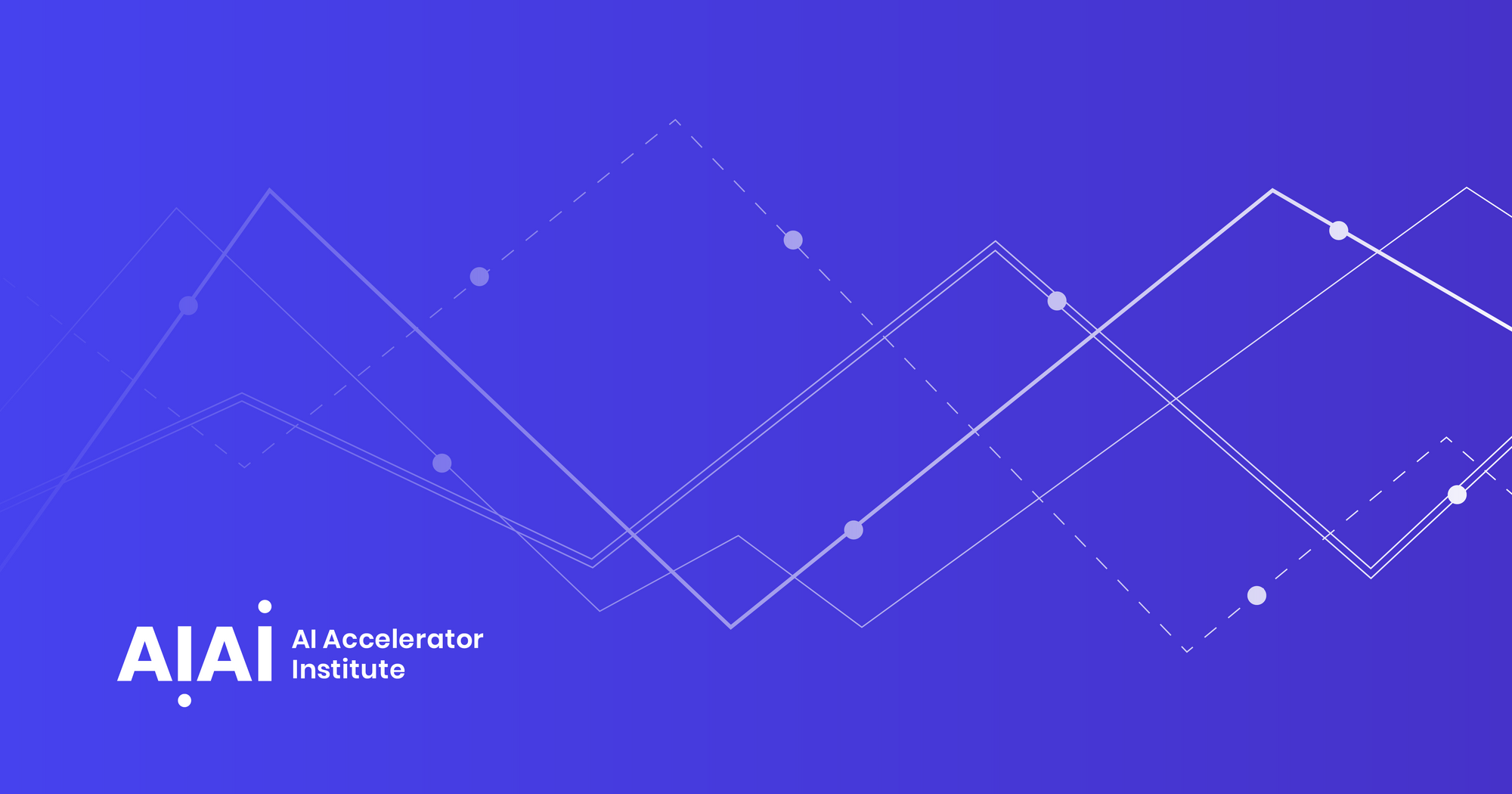
Rule-based segmentation vs machine learning
Marketers have been using personalization in their direct marketing strategies for a long time and in many forms. From a “welcome back” message for returning users to more individualized product recommendations in email newsletters, it is a known fact that customers who experience a more personalized shopping experience are more likely to spend and keep returning, whether it’s B2B or B2C.
Rule-based segmentation is a more simplistic way of personalizing marketing efforts than using machine learning. It uses rules determined by “if-then” scenarios, such as if it detects a website user from a specific location, it shows nearby events to that person. Or it might divide users by demographics such as age and gender and push marketing campaigns geared towards those groups.
But using rule-based systems takes a lot of work to add in all those variables manually, and many incorrect assumptions are made about customers. Marketers may pick a variable they believe to have the most significant impact on decision-making when it might actually be far from reality. With all the data marketers have access to, more advanced techniques are needed to use it to its full potential.
Personalized marketing is now considered a standard service, with 72% of consumers claiming they only engage with personalized messaging, so it’s only a matter of time until it becomes the norm for B2B too.
Enter machine learning. You feed the algorithm your data which is then used to determine patterns. This type of marketing goes far beyond rule-based personalization, as the algorithm can define segments in a way that humans simply can’t or wouldn’t have time to. Machine learning can analyze each traffic segment and provide the most relevant content to different audience groups. As a result, personalization can exist for every single visitor, and it can be done automatically.
Machine learning applications in direct marketing
So we’ve seen in a nutshell what machine learning can do for marketing personalization, so here’s a closer look at the specifics of how marketers can use machine learning in their campaigns.
Optimized advertising
Advertising is an essential avenue for marketers. Traditionally, it’s optimized manually using decision-making tactics like looking at which advertising channels to use, the timing of the ad, and the duration of an ad campaign.
Human limitations restrict this manual process, so machine learning can improve the performance of marketing campaigns by reaching more relevant audiences at the right time. With its predictive capabilities, machine learning can effectively optimize the likes of email campaigns throughout a customer’s life cycle to maximize any KPIs, optimize conversions and improve your ROI.
Predictive targeting
Deciding which customers to focus marketing efforts on can be a time-consuming and tricky process. To do it manually, marketers need to segment their digital traffic, launch A/B tests, wait for the results, and break them down, analyzing the performance of each segment which can then be used to manually set up a personalized experience for each of those segments.
It’s no wonder true personalization is so difficult to achieve. Machine learning takes away these time-consuming steps to predict the optimal setup, providing marketers with exactly which traffic segments they need to target and how to target them, whether with email, phone calls, or social media campaigns.
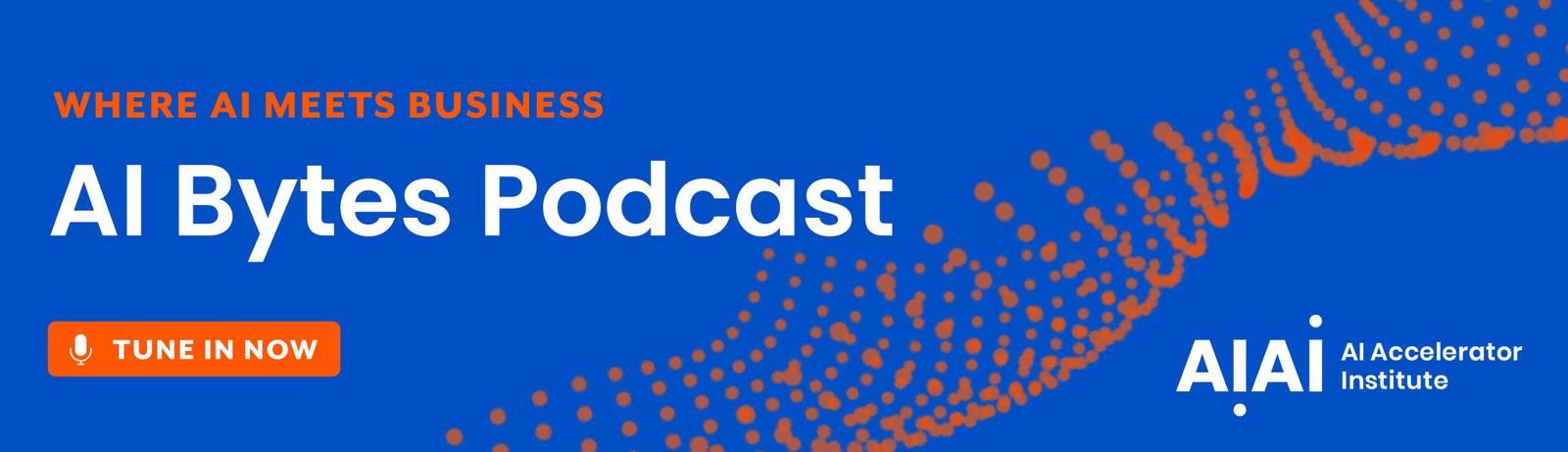
Automated email marketing
Machine learning can completely personalize email campaigns with ease, leveraging customer segments, content, and data about prospects. For customers to engage with marketing emails, they must resonate with them and be sent at the right time. Machine learning can predict which kinds of emails will perform best and send them out automatically, depending on a customer’s activity history.
The algorithm can learn which types of emails work best for each customer, using behavioral cues instead of simple demographics. These nuanced behavior patterns can potentially be turned into new and distinct marketing segments to be targeted with customized campaigns.
Customer lifetime value forecasting
Customer lifetime value (CLV) refers to the amount of money a customer is expected to spend on a business during their lifetime. It’s an important metric to consider because it helps marketers decide how much to invest in acquiring new customers or retaining existing ones.
Machine learning models can predict CLV based on a customer’s previous buying behavior and other demographic information and predict the future value of new customers. This information can be used to identify customers who are more likely to spend than others, and direct marketing campaigns can be tailored specifically to them.
Case studies
Still interested to learn more? Here are some real-life examples of how machine learning is being used right now in the B2C world to inspire us B2B marketers:
Netflix
As the world’s biggest streaming service, Netflix has over 160 million subscribers in 190 countries, which is an awful lot of data to work with. Netflix invests heavily in machine learning to improve its recommendation algorithms for users. Machine learning helps shape what programmes and films are shown to specific users, giving a personalized experience to every customer.
eBay
The e-commerce platform eBay utilizes machine learning to help them bypass the language barriers of their users. To connect international buyers and sellers on the platform, it brought in a machine learning system to translate product titles, descriptions, and reviews. This kind of system increased their overall commerce, and eBay’s Senior Manager of Machine Translation Science has said that it can “connect global customers” to provide the best experience.
Amazon
Amazon has been a big proponent of machine learning for many years to better understand their customers and provide personalized shopping recommendations. But more recently, the e-commerce giant has been using algorithms to make more sustainable packaging decisions.
Using product data and customer feedback, Amazon implemented a machine learning system to eliminate waste by identifying products that can be shipped in paper bags or folded into smaller packages. By 2020, the system had reduced the use of boxes from 69% to 42%.
Final thoughts
Machine learning has the potential to transform how we market to customers, and it will only keep getting more advanced as time goes on.
You can utilize the power of machine learning to remove the repetitive, time-intensive, mundane tasks that many marketers find tedious and focus on those more critical tasks that require the human touch.
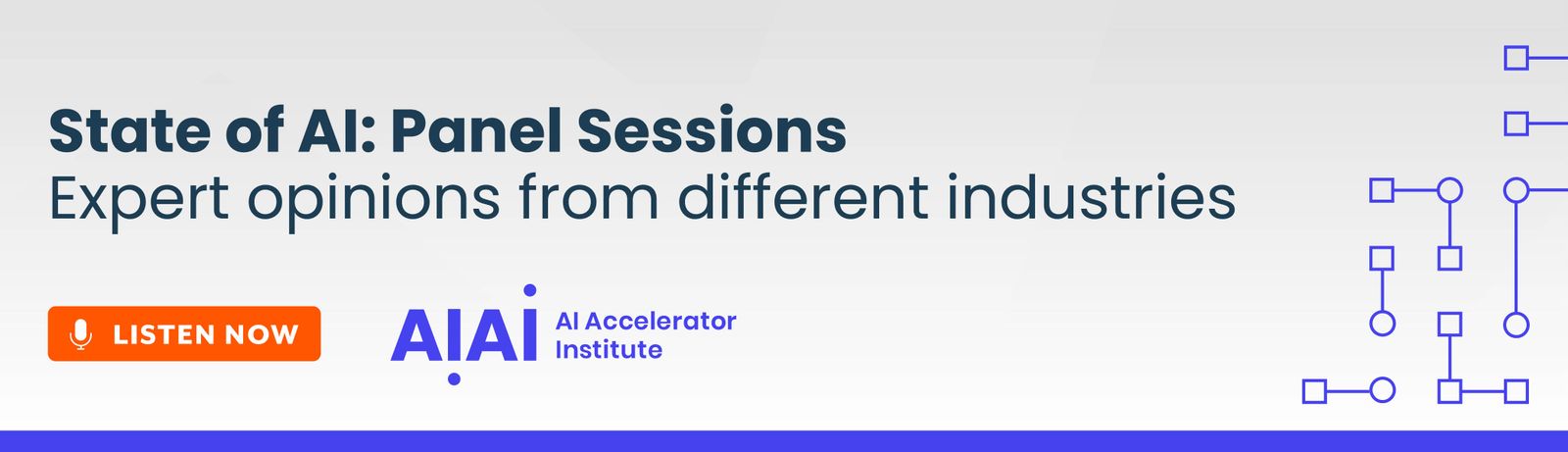