Artificial intelligence in manufacturing is a big factor in how operations are developing. AI can receive a mix of data from machines, people, and sensors and apply it to specially designed algorithms that help to optimize operations.
AI is behind a massive change in the industry, from remotely monitoring machinery to identifying problems before they happen, helping to minimize waste in the supply chain.
Did you know that in 2020, around 60% of manufacturers were using AI to reach better speed and visibility across their supply chain, improve product quality, and optimize inventory management?
The manufacturing industry generates massive amounts of data, but can also waste massive amounts of data. Through using AI, businesses can leverage big data to its full potential in order to improve their decision-making.
In this article, we’ll take examine AI in manufacturing, namely:
The importance of artificial intelligence in manufacturing
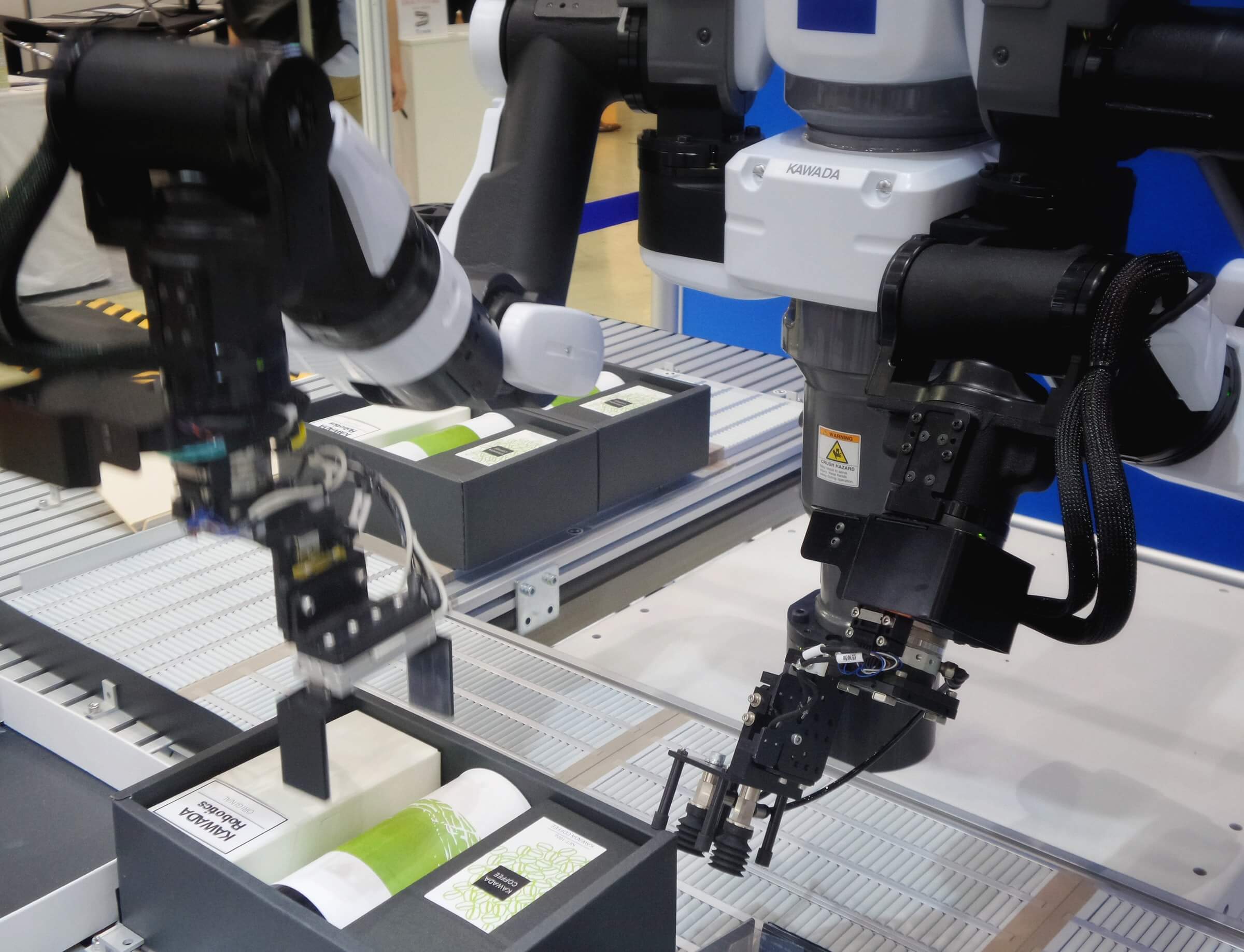
In product optimization, AI and machine learning, in particular, can be powerful tools in minimizing the use of additives and energy in industrial processes. Traditional methods still have a certain degree of uncertainty as part of complex chemical processes, with extra quantities of pricey additives being used to cover deviations in processing.
AI can step in and provide the exact quantities needed to help prevent a costly surplus with every process. It can let businesses build a model that receives data from multiple sources like the quality of raw materials and material composition, all from hundreds or thousands of sensors. This trained model can then predict with extreme accuracy the final product parameters and calculate the exact amounts of additives needed for each batch.
Similarly, limiting downtime and maximizing the effective operation of production lines is something AI can help with. A machine learning model can monitor specific activities for anomalies or errors that point towards specific issues with machines. It will then use predictive intelligence to consider whether a human employee needs to take action. AI can also be used in order to predict whether machine parts need replacing and what needs to be ordered. This leads to reduced downtime and the prevention of expensive inventory piling up without needing to be used.
The models aggregate massive amounts of data to recognize important patterns and trends that may lead to parts failing and even lead to predictive maintenance for more efficiency. As the models learn, the more accurate and precise they become.
Use cases of artificial intelligence in manufacturing
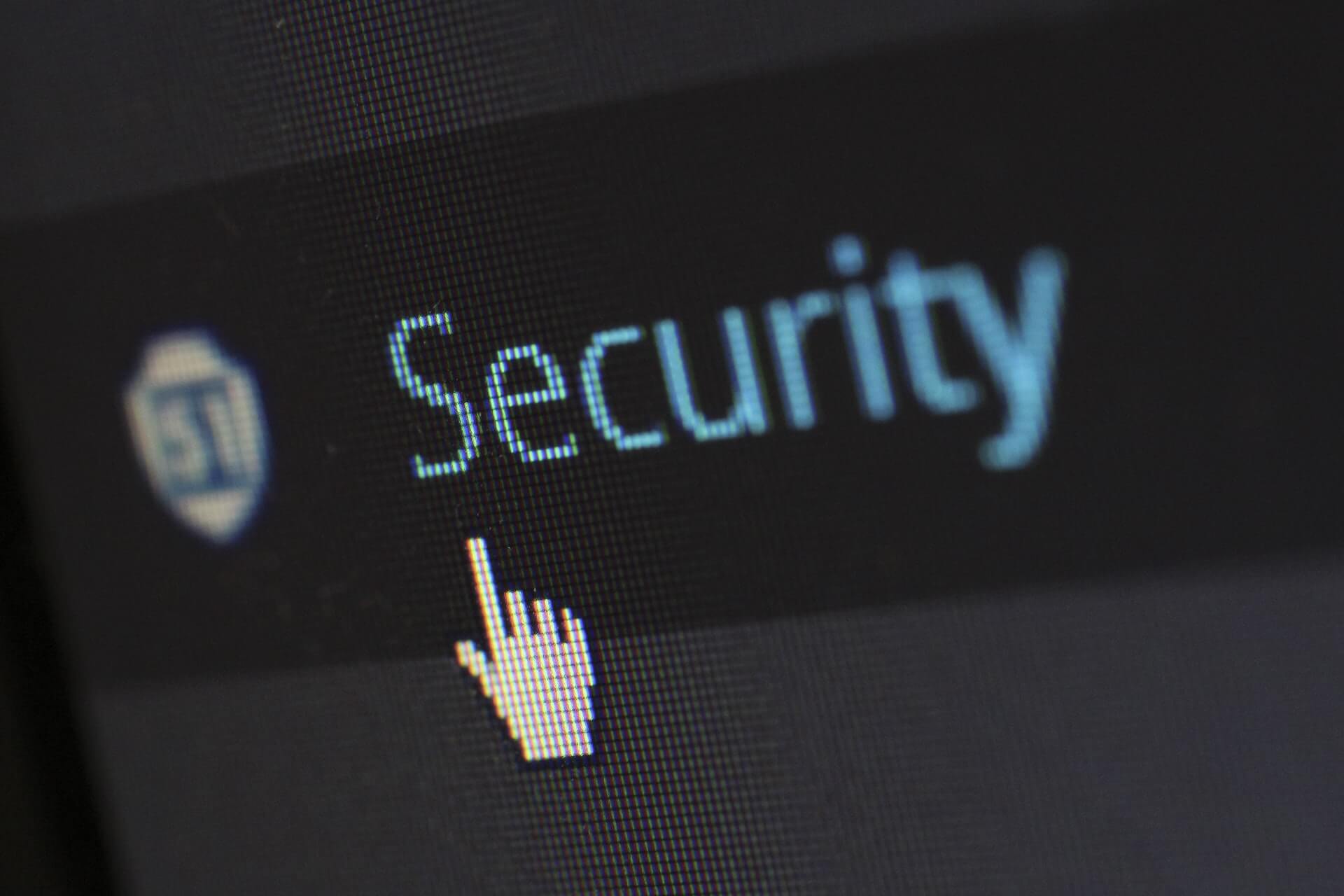
1. Computer vision
AI and computer vision are facilitating production, logistics optimization, fleet management, and quality inspection. Machine vision can help to increase capacity and reduce transactions in logistics and warehouse areas. This can translate to the elimination of scanning needed by human employees or by making sure that customer orders are packed as accurately as possible.
Dash cameras are another example of machine vision. In fleet management, these cameras can often include options such as in-cab monitoring.
2. Predictive maintenance
Pinpointing potential errors and downtime by analyzing sensor data helps manufacturers to predict when machines will stop working and schedule maintenance before it happens. This leads to improved efficiency, as operations don’t need to stop, and to reduced costs from machines failing and needing to be replaced. Businesses can also automate visual inspections with computer vision in order to save potential losses of up to 20% annual sales.
3. Cybersecurity
With manufacturing and operations technology environments generating big amounts of data in security logs, filtering normal day-to-day actions with suspicious ones can be a massive undertaking. AI can autonomously detect fraud, intruders, malware, and much more, helping to tackle modern cybersecurity threats and challenges at a faster and more accurate rate than a human employee.
4. Edge analytics
Offering decentralized and fast insights from datasets, edge analytics collects data from sensors or machines. This data collected on the edge can then be analyzed and transformed into insights that can be used to optimize operations. Edge analytics can then:
- Track employee health and safety
- Improve both production yield and quality
- Detect the beginning stages of performance deterioration and risk failure
5. Robotics
Manufacturing robots are excellent to automate repetitive tasks and help eliminate human employee mistakes. From welding to product inspection and assembly, these robots let employees shift their attention to other areas.
Adding AI into the mix, we’re left with robots capable of not only monitoring themselves but also training themselves to get even more efficient. And with computer vision, robots can accomplish precise operations.
6. Inventory management
Due to their capacity for forecasting and planning supply, machine learning solutions can be used to predict results more accurately. This will enable businesses to manage their inventory by planning when products need to be replenished, offering more accurate arrival times for goods. AI can also create models to predict future demands based on existing inventory data.
Benefits of artificial intelligence in manufacturing
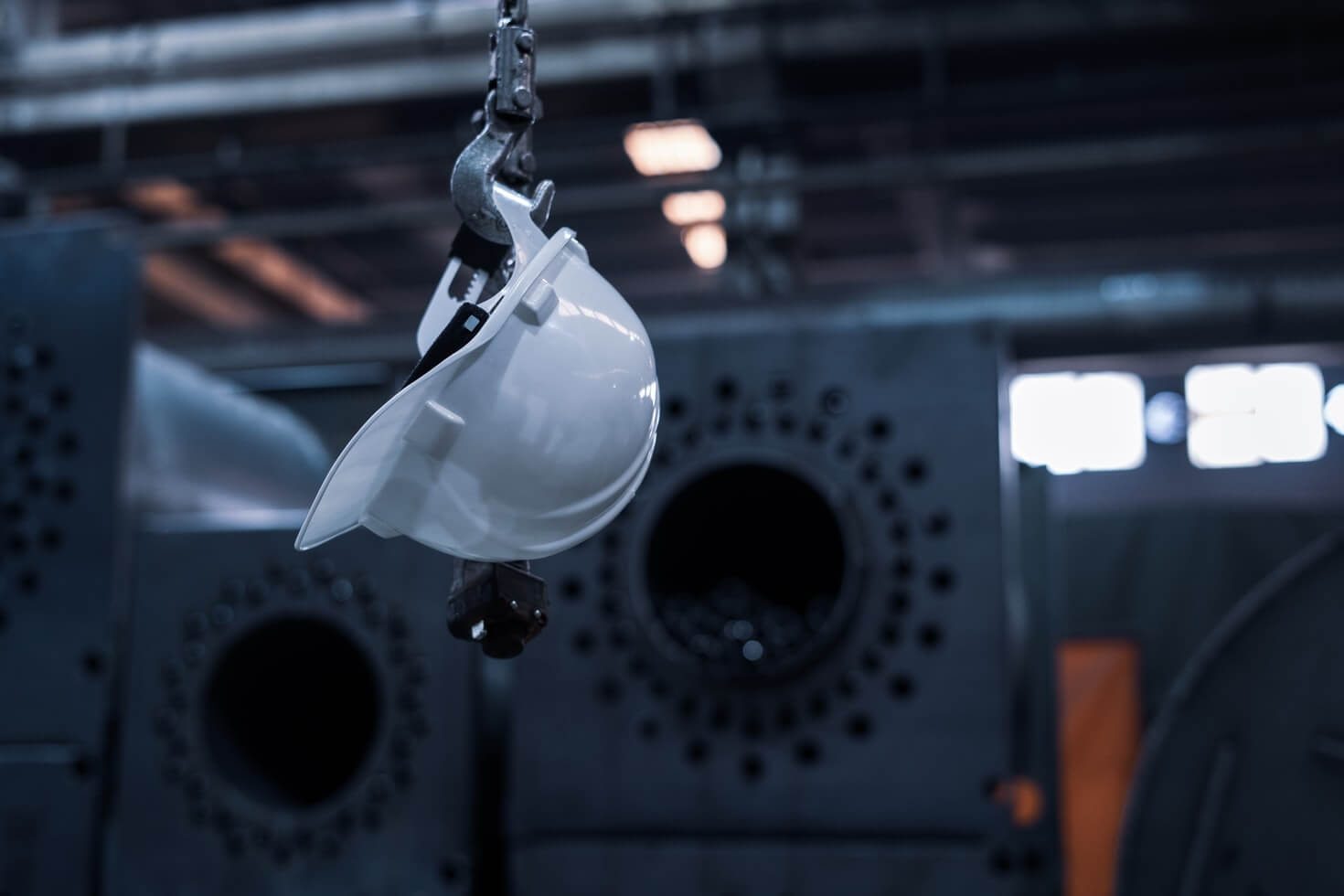
Safety
Human employees are fallible - particularly when distracted or tired - which can lead to accidents and errors happening on a factory floor. AI can minimize, if not eliminate, the occurrence of accidents and errors. Having remote access control leads to a reduced need for human resources, and advanced sensors integrated with IIoT (Industrial Internet of Things) devices help make the installation of barriers and other safety guards more efficient.
Lower operational costs
Although potentially requiring costly capital investment, the ROI of adopting AI in manufacturing will increase with time. Predictive maintenance, for example, can greatly reduce the costs involved with downtime and broken machines.
Machine learning and CAD, combined with advances in 3D printing and IIoT connected devices, allow businesses to test products virtually and print a less costly prototype before the final version is mass-produced. This minimizes the costs involved with trial-and-error.
24/7 production
Unlike human employees, machines can work around the clock without needing sleep. This means the production line can be working 24/7, expanding the production capabilities to meet increasing customer demands. Machines are also more efficient in areas like assembly, reducing turn-around times.
Direct automation
IoT devices integrate manufacturing processes alongside big data, making them programmable through a logic controller. This leads to data that is generated, recorded, and analyzed, covering all processes in production. When programmable logic controllers have an AI capacity for deep learning, they can automatically react to the data and take action in real-time without requiring human employees to intervene.
The future of artificial intelligence in manufacturing
With the intention of raising the productivity in the UK manufacturing industry by 30% by 2030, the Manufacturing Made Smarter challenge is run by Digital Catapult to see 14 startups addressing the UK’s prevalent challenges in manufacturing. Funded by UKRI (UK Research and Innovation), the £147 million investment will be matched by a minimum of £147 million from the manufacturing industry.
It will help support both technology developers and manufacturers in proving their ideas, scaling ‘outside the box’ digital technology solutions, and quickly develop with experts. By helping to remove the risk out of innovation and supporting collaborations between a variety of UK manufacturing sectors, this challenge will impact digital technologies like:
- Connectivity (5G, LPWAN)
- Automation and robotics
- AI, ML, and data analytics
- Virtual reality and augmented reality
- Additive manufacturing
- The IIoT (Industrial Internet of Things)
These technologies will be applied to projects such as:
- Adaptable and flexible manufacturing operations and skills
- Connected and versatile supply chains
- Smart, connected factories
- New easy to test, design, and make products
Through this investment, the goals are not only to raise the UK’s manufacturing productivity by 30% in the next four years, but also to help the UK shape the future of the industry, accelerate the drive to net-zero emissions, and create thousands of highly skilled jobs.
Ascalia, working alongside Sainsbury’s, is working towards finding a solution to microbial control in ready-to-eat foods. By using AI and high-tech cameras, it’ll make sure food is safe from both microbes and rotting. Singular Intelligence is also working with the supermarket to make use of AI-based decision-making in order to develop a predictive shelf-life model for its supply chain, including an automated and centralized optimal control system.
Final thoughts
AI can have a great impact across the entire chain in manufacturing. Using AI tools to pinpoint opportunities in improving operations helps businesses deliver their top and bottom-line objectives, alongside driving success in future decisions.
Want access to fantastic talks with AI experts from industries like manufacturing, healthcare, retail, and more? Sign up for an AIAI membership today!
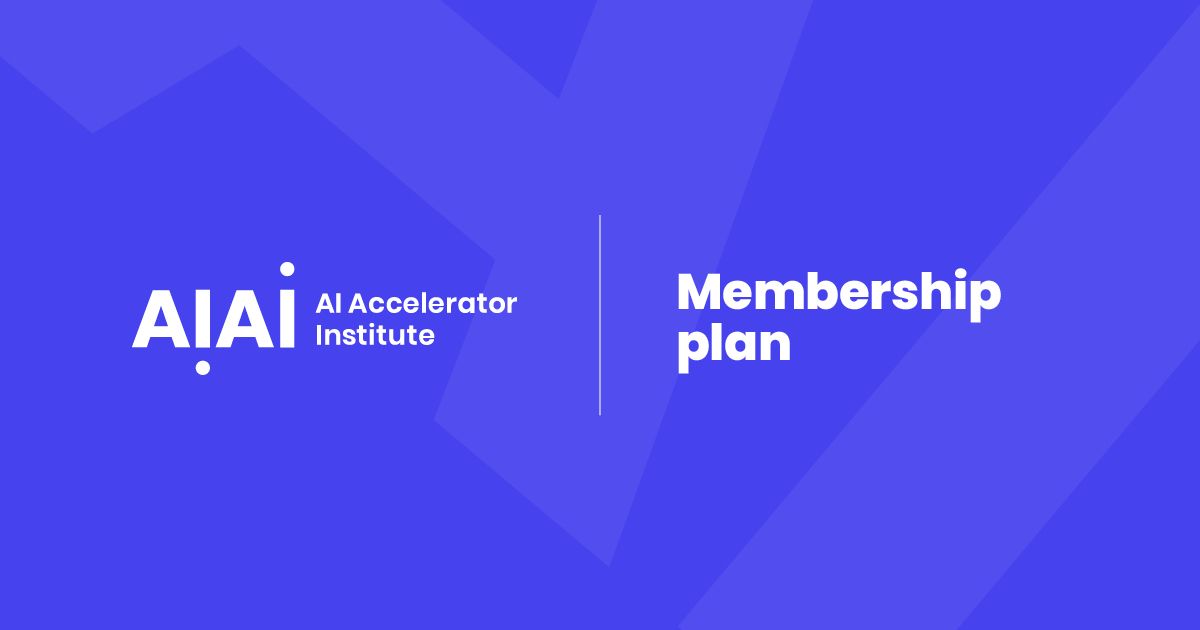