With AI reshaping all industries, it’s no wonder that it’s having such a big impact on healthcare. From natural language processing (NLP) to machine learning, and deep learning, AI is helping medical professionals to identify patient needs much more accurately and faster than ever before.
Through analyzing the large amounts of data created in healthcare, algorithms learn to identify and label patterns in the data. There’s also the potential to lower costs and personalize care for patients, however, concerns over prejudice and discrimination exist.
In this article, we’ll take a look at AI in healthcare, namely:
- Best practices
- Its applications
- Benefits
Best practices for AI in healthcare
1. Transparency and understanding with AI-driven recommendations
There needs to be transparency about how an AI system generates recommendations so that algorithms can be adjusted. Medical professionals who use AI also need to consider the possibility of bias in data analysis, as the analyzed data is often just from patients who have already been seen.
The lack of data from populations that are underserved can severely skew results. This means that continuous learning AI systems are likely to need subjective human employee evaluation to make sure these systems are still providing the results they need to provide.
2. Accountability and liability
Privacy laws, governing bodies, lawyers: These are all vital for AI in healthcare. Its impact has preceded both regulators and legislators, which means that the application of ethical principles is needed while we wait for the industry to catch up.
We need strict laws applied to AI to restrict the release of medical information, including subject matters not necessarily covered by privacy laws but existing in AI, such as genetic testing.
3. Consider limitations and accuracy
Separating correlation from causation is important to recognize when the algorithm's output is impacted by disparity. Health algorithms can have a racial bias, as demonstrated when a health system utilized a common AI algorithm.
When trying to predict which of the patients needed follow-up care, this algorithm found that 82% of the patients were white, while just 18% were black, as it assumed that higher spending in healthcare correlated to worse health. While this algorithm has since been fixed, it highlights how disparity can accidentally be present in AI.
AI applications in healthcare
Healthcare management
- Fraud detection
- Market research
- Operations
- Brand management and marketing
- Customer service chatbots
- Pricing and risk management
Research and development
- Device and drug comparative effectiveness
- Gene analysis and editing
- Drug discovery
Patient care
- Real-time prioritization and triage
- Personalized medication and care
- Automated prescription and diagnosis
- Patient data analytics
- Surgical robots
Medical imaging and diagnosis
- Medical imaging insights
- Early diagnosis
Benefits of AI in healthcare
Improving imaging analysis
AI can be used for case triage, helping medical professionals review scans and images and identify important insights that allow them to prioritize critical care cases. This also leads to a reduction in potential errors when reading electronic health records (EHRs), and more accurate diagnoses.
When conducting clinical studies, there’s often a huge amount of information created - all of which needs to be analyzed. AI can very quickly analyze this data and compare it to other clinical studies to find patterns and make connections.
AI tools can look over medical histories, lab results, and past diagnoses to deliver context behind imaging that can help radiologists and other professionals have a more accurate diagnostic process.
Forecasting kidney disease
A report from the journal BMJ Quality & Safety, from 2014, found that around 12 million people in the United States are affected by medical diagnostic errors every year. Certain diseases, like acute kidney injury (AKI), for example, aren't always easy to detect - but often cause patients to very quickly deteriorate. AKI is a dangerous complication that can occur after cardiothoracic surgery, and it’s important to predict it early.
The U.S. Department of Veteran Affairs (VA) in partnership with DeepMind developed a machine learning tool that’s able to predict AKI up to 48 hours in advance. This tool was accurate in over 90% of cases, in comparison to traditional care methods.
Conducting predictive analytics
AI in banking, for example, also uses predictive analytics to detect patterns and make correlations. Natural language processing and machine learning in AI-driven predictive tools can read through EHRs in real-time to make connections between patient symptoms and family medical history. These tools can catch an illness before it becomes life-threatening by predicting chronic illnesses and tracking their progression rate.
This allows medical professionals to create insights that fuel data-driven decisions, which in turn improve patient outcomes. This can be applied to various illnesses like strokes, congestive heart failure, liver cancer, and more, leading to lower patient costs thanks to an early and accurate diagnosis.
Analyzing unstructured data
Medical data can be stored as unstructured data, making it difficult to both access and analyze. Through the implementation of AI, it’s easier to search, collect, store, and analyze medical data in any format. AI can also help with repetitive tasks and creating treatment plans that are accurate and tailored - much quicker than searching, identifying, and analyzing data from paper-formatted EHRs.
Machine learning technologies can scan all the biomedical and EHR data to offer reliable and fast answers to medical professionals.
Want to know more about unstructured data? Have a look at the article below:
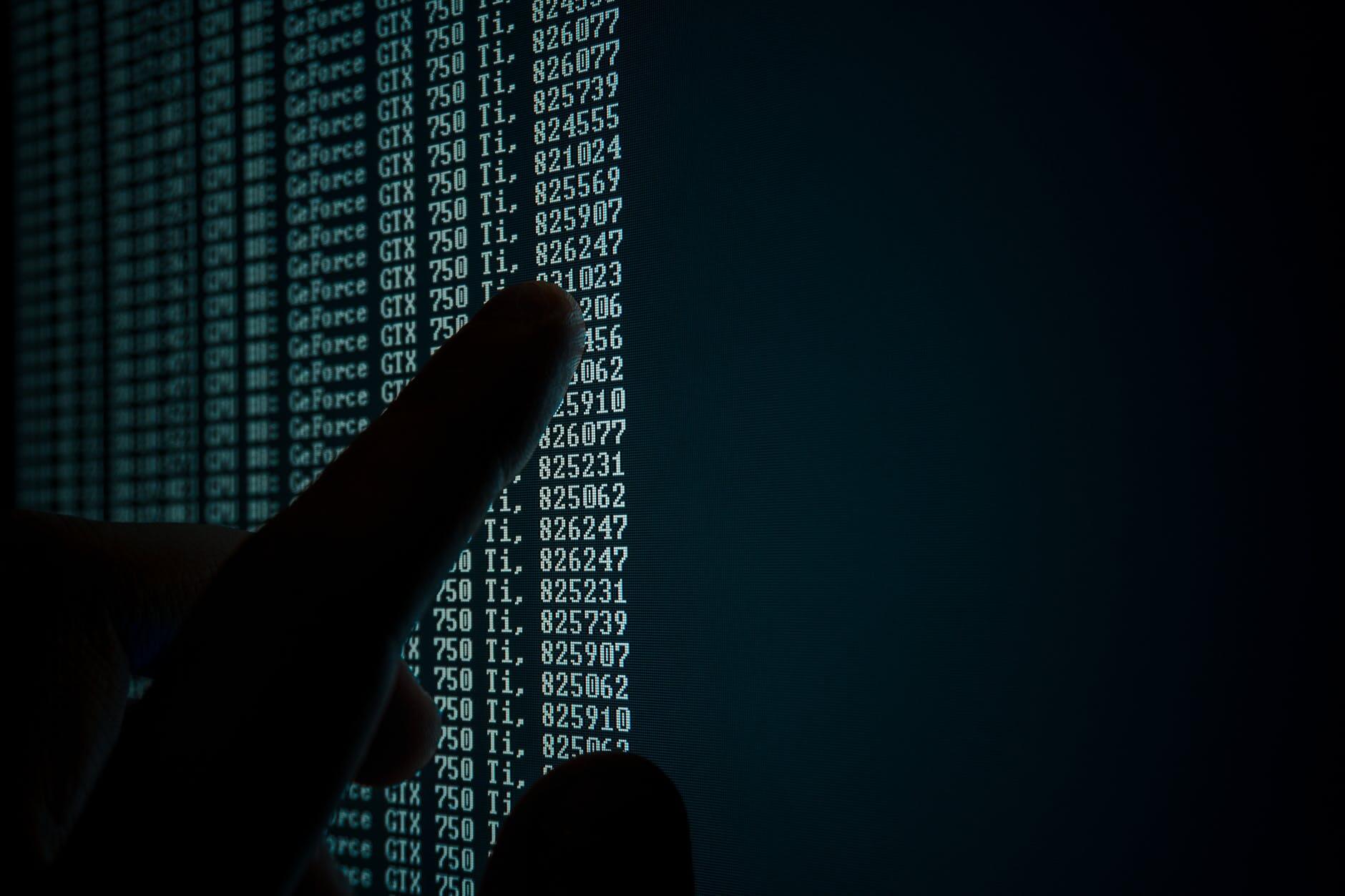