AI Bytes delivers digestible intelligence from the world's leading AI in business practitioners, offering a close look at the lifecycle of AI vision, strategy, business objectives, and much more.
Podcast host Ashok Ramani sat down with Daniel Bruce, CPO at Levatas, to discuss his expertise and insights in AI from his 20-year long career.
In this episode, Ashok and Daniel discuss:
- How Levatas helps their customers
- How humans and AI can collaborate together
- AI use cases
And more below:
Q: Hey, Daniel, welcome to the AI podcast. Excited to have you here.
A: Thanks. It's great to be here.
Q: Awesome. This is great. So, Daniel, you are the Chief Product Officer at Levatas. You've been in the tech industry for 15 plus years, and you've seen the evolution of big data analytics and AI. Right. So, tell us your story, your journey, and what have you actually seen happen with AI?
A: Yeah, I've been in the industry for about 20 years now. I joined my current company, Levatas, almost 15 years ago now. Levatas really started as a customer solutions provider at the time. We would provide websites, we would provide custom mobile applications, and enterprise applications.
We really cut our teeth on a very broad range of technology offerings. In the last 10 years, we’ve really started pivoting as an organization around artificial intelligence. Part of that was personal for me. It’s always been an area that I'm very passionate about.
Part of it was just seeing the need in the industry from the customers that we're working with. They were excited about this new technology, but people just didn't know what to do with it. And so through the years, we've really shifted and started to refocus as an organization.
We’re very tightly focused on computer vision, artificial intelligence, and natural language processing. Where we are today at Levatas really has a tight specialization and focus around automated inspection solutions that make up a significant portion of our business.
It's just been fun! It's been a fun journey, seeing the industry evolve, helping our clients navigate the hype and fear cycles that come along with a technology like this. And it's just been fun to be part of the ride that so many organizations are a part of.
Q: Daniel, you've been in touch with customers, right? Do you consult with them? You help them with strategy, you help their implementation, and the lifecycle, right? So, tell us what are you seeing right now? Is there a lot of hype?
A: Just over the last decade, we've seen quite a broad range of solutions. AI is really revolutionizing every industry it touches. Whether it's healthcare, government operations, logistics, transportation, self-driving cars, every industry is really right in the evolution now!
For Levatas specifically, we really focus very heavily on the space of automated industrial inspections. What that typically looks like for us is an industrial setting. It could be manufacturing, construction, or logistics, but a lot of big organizations have specialized, highly trained, and highly paid individuals who are performing manual inspections within a facility.
That might take the form of taking a quick look at equipment that's overheating. Looking for an analog gauge in a plant that isn't digitized and needs to be tracked to make sure that equipment is operating normally. A general use case would be just looking for anomalies.
In general, in an industrial context, change is a bad thing. If everything is functioning normally today, but tomorrow, something looks very different, that's generally something of interest to the plant operations folks. Our goal with our customers is really helping them free up some of the time that's required from those human operators. This is instead of doing manual operating rounds, which can be boring and difficult, and tedious.
Our goal is to free that up. So, these inspections can be performed much more frequently, with a higher degree of accuracy. Let humans focus on what humans do best. Typically, the use cases at the business level involve increasing safety for humans, increasing the liability for equipment or plant operations. Ultimately, putting dollars back on the bottom line for these organizations is allowing them to reallocate their savings to other areas of their organization.
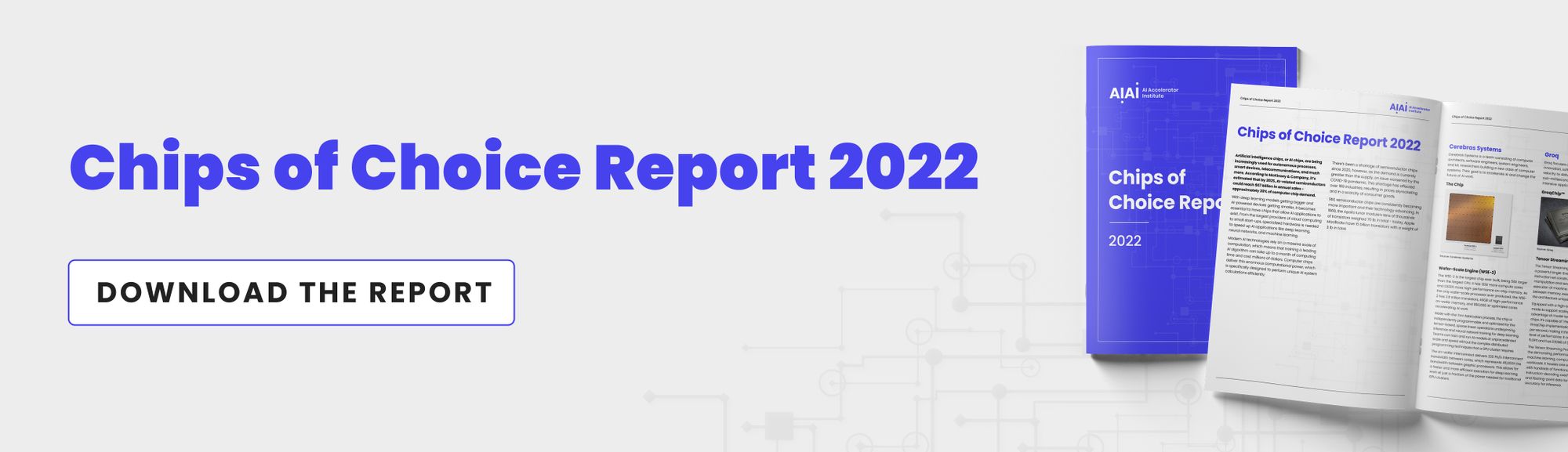
Q: How can humans and AI come together to collaborate?
A: I think the goal ultimately of artificial intelligence is to mimic human intelligence in some meaningful way, usually around specialized tasks. It's easy to take a sort of naive, or an incorrect perspective on how human intelligence works in the first place.
If you think about what makes humans intelligent, we don't typically gain intelligence in a vacuum. We don't learn language, we don't learn math, or writing or language or tasks that we do every day in a complete vacuum. We're usually partnered with other humans, often friends, family, teachers, parents, who are alongside us.
And so when we think about artificial intelligence, I think it's probably faulty thinking to think about dropping an AI model that's just going to function in isolation from human experts. You can’t just drop an AI model in there and let it do its thing. That's very dangerous, and probably a naive perspective.
Now, certainly, that's possible in some very trivial artificial intelligence use cases where there's not a lot of judgment needed. But in cases where there's a lot of judgment required, we believe in pairing human expertise with artificial intelligence models to deliver a better result for the business.
AI can deliver some training wheels for the AI model so it can learn to mimic the way that humans learn in the first place. Our solutions offer this human-in-the-loop approach where an AI model can alert a person to an error and a human operator can then use their judgment to look closely at an issue. We do need the benefit of human experts there to help. And in the meantime, we can allow models to get smarter and smarter over time.
That is really a core tenant of our approach in industrial inspections. It's certainly not applicable to every area of AI in the same way. In healthcare where people's lives are on the line, the stakes are different than if an AI makes the wrong call, maybe a piece of equipment stops working and needs to be repaired. There are certainly different ways that that can be applied. But in any case, we see that human and AI collaboration is absolutely critical.
Q: We want to be in a position where the AI is not really replacing humans but is actually learning from the humans, right? That's very cool. But when it comes to some AI use cases, there is tons of data to the point that it's too much noise! But then you can also have this sparse data problem, right? Not enough data. What kind of technology are you adopting in AI in deep learning? Can you talk about that, Daniel?
A: I think that's a critical question. It's certainly something that's really at the heart of the industry right now. So I'll address that at the highest level. I'd say there is a much-needed industry trend away from perfecting models, which has been the trend.
We’ve been working very hard in the last decade to get better models, faster and higher performing models. There's a very much-needed trend in the industry now towards focusing more on the data than on making the model better. Time spent getting higher quality data, or even a higher volume of data is probably a better way to get a better performing model than trying to get a 1% performance improvement on the model. That's certainly true of the industry at large. And it's refreshing to see some of the leaders in this space really pushing that direction and that narrative.
Q: Yeah, I’ve read about organizations actually starting a movement around data, right? Enough of the models! We have to start looking at the data, because that's kind of where you have the exponential improvements. Do you agree?
A: Yeah and one of the challenges is when your data is bad, or when you just don't have enough data. It can create this vicious cycle where you’re actually overfitting your model around bad data. Every incremental bit of improvement, or perceived improvement, is actually not improving your model. It's a perceived improvement, but only because you have a limited data set to work with.
It's like preparing for a test where there's only three questions on the test. And the more that you prepare to get those three questions, are you really getting smarter? No. You're just getting better at answering those three questions.
These deep learning neural networks are phenomenally good at memorizing information, even very large quantities of information. That means when you have a massive data set and a high-quality data set, you can take this deep learning network and get really, really impressive results from it.
The downside is when you've got a small data set, your model can literally memorize the entire data. But it tends to be very abstract unseen data. That's the reason that you build these models. It’s to be able to predict unseen data. That's the first layer that is certainly a trend in the industry.
In this space of visual inspections, there are some unique characteristics of image in visual classification, that really helped, they really helped mitigate that challenge. So in general, it is possible in an image classification scenario to start with a relatively modest data set.
With a small data set like that, we're able to actually get some quite impressive results with a relatively small set of data. For anybody listening that isn't familiar with it, it's a little bit like learning how to play an instrument. Let's say you learn how to play the piano by practicing one piece of music over and over again.
You get your technique down, you learn how to read the music, and you get all of that down. And then you take everything that you've learned, and you transfer that over to a new song, or a new piece of music. That's very much how transfer learning works. And it works.
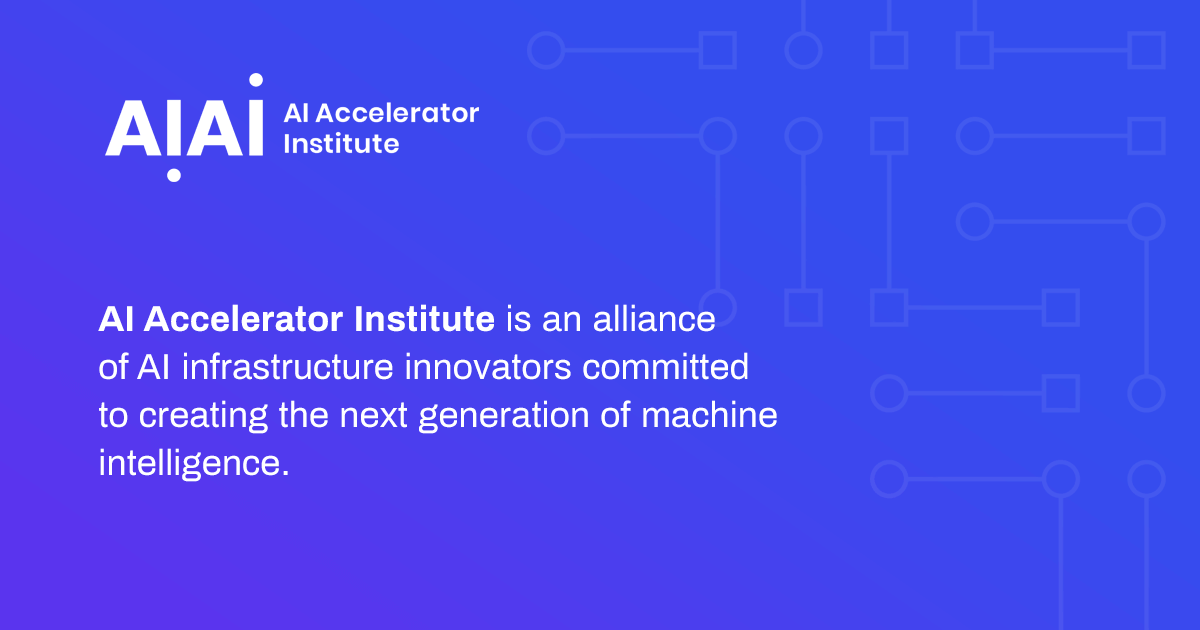
Q: There's an emerging trend around using things like active learning, right? How do you actually use that?
A: So, when I talk about ‘human in the loop’ learning, that really that's another way of saying ‘active learning’, which is really allowing a model to ask for help and ask for training on the fly. It’s allowing that model to rewire itself.
We see that as a critical part of a lot of these use cases. And it's particularly true in an inspection space. So, if you think about inspections, for example, take the use case of identifying a piece of equipment that is faulty, there may be 1000s, or even 10s of 1,000s of different fault states. They are relatively rare.
Imagine going to a customer and telling them you want to build a model that helps you identify all of these fault states. All you need is to create a data set that shows all 10,000 fault states.
What's required is an active learning approach where you train a model to understand what normal looks like. We're going to train it on some subset of what types of faults it might encounter in the real world, based on what data is available to it.
When this model sees something that it doesn't have a high degree of confidence in it needs to be able to send an alert that it hasn’t seen this. “Take a look, tell me what to do with this next time” You also want that model to collect that feedback over time. Maybe the next time it sees a failure that looks like something it was trained on before, it’s able to perform better at that point. That's the goal, it’s to completely close that loop.
Q: Awesome. You talked a little bit about active learning, right? When you actually advocate for an AI lifecycle, what kind of pitfalls and gotchas do you need to look out for? What do customers need to watch out for?
A: I’ll use the analogy of a parent. As a parent, you're watching your kids grow up and learn things for themselves. The first time you send your kid off to school, you ask yourself, do they need to know how to handle every situation that's going to come up? As parents, we have a tendency to worry about that a little bit too much, right?
We underestimate the resilience of our kids. It's very much like that as a model developer. We're like very proud parents. But we don't want to let go of our kids until they're perfect. So, the first challenge there is just recognizing that just like a parent, if you hang on forever, your kids will never be perfect.
As a data scientist, if you hang on to your models too long, they will not get to be perfect because they have to experience the real world. They have to fail in a controlled context, to be able to learn how to handle that. I'd start just by saying, the first pitfall we find is customers or partners or data scientists who just won't let go until things are perfect.
We all often find ourselves just needing to explain to customers, that's not a realistic standard. People who are succeeding with AI are okay with some degree of imprecision, some degree of mistakes, because guess what, your people who you trust and you put a lot of faith in making mistakes, too.
That's how we learn. Don't set that kind of unrealistic expectation. I think because of the way that traditional software works, people set expectations that machines always do things right. But the reality is, our goal is to replicate with AI that human judgment and learning process. That requires having comfortability with shipping and knowing it's gonna make some mistakes sometimes. How do we educate the humans that are going to be interacting with this? How do we educate them to be good partners with technology? Pairing the two together and setting realistic expectations and then rolling it out in a controlled way where that initial failure and initial learning is constructive and manageable.
Interested in joining a network of over 3,500 AI practitioners? Then pop over to the AIAI Slack community and start sharing insights and expanding your connections today.