Computer vision is just one of artificial intelligence’s (AI) many facets. It helps computer systems to better understand the world and detect objects within it. With algorithms being able to understand both images and videos, computer vision is propelling a variety of innovations like autonomous industrial machines and self-driving cars.
With the market value of computer vision technology estimated to hit US$48 billion by the end of 2022, we can only imagine how many more exciting things we’ll see.
In this article, we’ll cover just a few trends in computer vision that we’ve spotted this year:
Interested in more computer vision topics? Why not sign up for our Computer Vision Summit in Boston, on October 20, 2022?
Edge computing
Edge computing helps to increase operation response time at high bandwidth, processing near the data source and not in the cloud. Computer vision constantly needs to consider how to better protect data privacy while processing data rapidly and in real-time.
And because edge computing doesn’t need a data center, it’s a great solution to computer vision’s data privacy issues. With a private architecture that makes it difficult for cyberattacks to succeed, edge computing can help to connect video management systems and cameras to quickly spot harmful activity and prevent breaches.
Uses in Healthcare
Computer vision is helping to correctly diagnose patients in healthcare settings. Medical imaging has greatly improved, with technology like Microsoft’s InnerEye software helping to detect tumors and other abnormal cells. It does this by reading 2D images and then rendering them into 3D.
Thermal imaging, for example, was essential during the COVID-19 pandemic. It used AI to create image/video sequences, detecting light-emitting objects. Thermal imaging helps to overcome the challenges of 2D inspection.
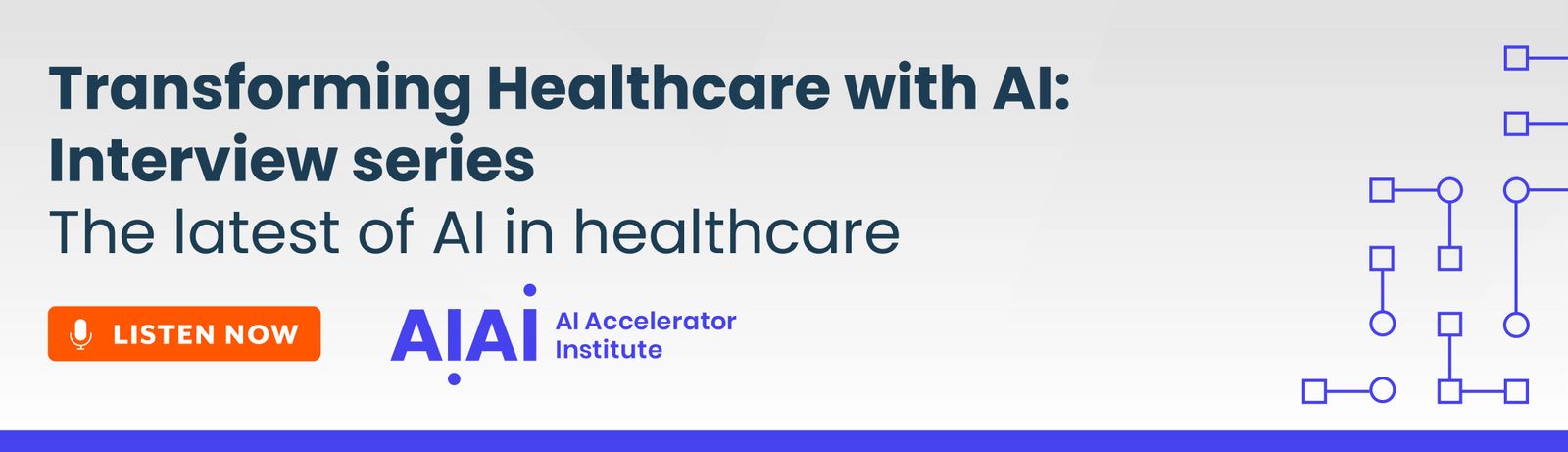
LiDAR technology
LiDAR, or Light Detection And Ranging, is a great technology for creating high-resolution maps, and it’s behind the rise of autonomous vehicles. The camera is placed on top of these cars and constantly spins to provide a 360° view.
As the camera spins, it uses ultraviolet light pulses so that it can detect objects in its surroundings. The pulses calculate the distance between the car and the range of the object by measuring how long it takes for the ultraviolet light to return to the camera.
LiDAR can also be used to make 3D digital representations and has applications in archeology, atmospheric physics, surveying, and much more
Interested in knowing more about LiDAR? Then get your ticket for the Computer Vision Summit and watch Zohaib Mian from Velodyne LiDAR give a panel on the technology.
Synthetic training
By using synthetic examples instead of real data to train computer vision models, you can accelerate their learning process without impacting their ability to react appropriately in real settings.
Graphics processing units (GPUs) have advanced to allow for high-fidelity rendering in mere seconds, so they can generate large volumes of quality training data with a high level of detail.
Explainable AI
Explainable AI consists of a set of frameworks and tools that better helps humans understand predictions made by machine learning models. The majority of explainable AI methods can be applied to any existing model without you needing to change them, however, they don’t give enough detail about its decision process.
By deploying machine learning solutions that are explainable by design, the models themselves can offer an explanation based on each prediction.
Neuro-symbolic AI
Neuro-symbolic AI’s goal is to combine today’s deep learning techniques with traditional symbolic AI methods, which tend to rely on rule-based reasoning. This approach allows for learning with fewer data and the creation of inherently interpretable models.