This talk was presented at AI Accelerator Summit San Jose 2023. To watch more insightful talks by AI experts, sign up for our membership and go OnDemand.
Hi, I’m Joshua Tamayo-Sarver, and today I'm going to talk about getting real change from artificial intelligence. What I'm hoping people can leave with, and what I'm hoping to see in this world, is that we can take healthcare, or just about anything which is deeply, deeply broken, add the power of artificial intelligence, and improve lives.
The purpose of this talk is for me to share my experience in doing that, or trying to do that. There have been mostly failures, but there's also been a smatter of successes in there over the past 15 years.
The first thing to remember is that this is actually important work, whether you're in healthcare or outside of healthcare. People don't get the care that they need. Now, whether that's because they're not getting the healthcare they need, or because they're busy doing things that don't give meaning to their lives, that's really where we can make a difference.
We've all seen people needlessly suffer. I know I have. And I think we all miss the people who are no longer in our lives. And I think we want to anchor everything that we do back to having some purpose.
My promise to you is that I'm going to share four things that I've learned from screwing things up over the past 15 years, with some successes in there. And with that, I hope that you're able to turn what I have, which are big, geeky dreams, into life-saving differences.
Deeply understand the problem
An effective solution starts with a deep understanding of the problem from multiple perspectives. I do a lot of consulting for pre-seed, to seed, to start-up, to the big four tech companies when they're getting into healthcare, and how to make a difference.
And the biggest problem that I’ve noticed is not understanding the problem. So I understand the problem with not understanding the problem at least.
Let's take a scenario. The problem may not initially be apparent, so let's go into that problem and then figure out how we can do even better than what’s on the surface and what's being done currently.
Let's say a friend and his son went to buy a car. That's a pretty good target for an AI. You go into the car dealership and say, “I want a car.” And you try and make it as quick as possible to go from, “I want a car,” to, “I have a car.”
You can automate a lot of the processes: the financing, the loan, the car selection, and all the paperwork. That's a typical consumer experience.
The goal is to go from “I want,” to, “I have,” as quickly and easily as possible.
But I actually think there are a lot of areas of our society where that's not really what we want to do. And I think this is a place where we can maybe move the needle forward a little bit.
In this case, the person wanted the car, and he got the car. But did he need that car? In that consumer model, we assume that ‘want’ is the same as ‘need.’ Is this an opportunity where we can actually intervene to say, “Is that really what you really, really want?”
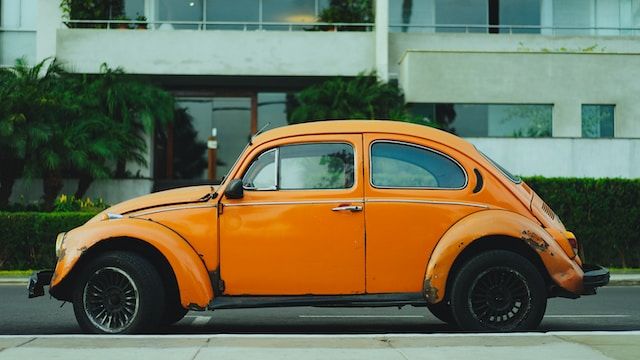
I think if I went into a car dealership, and the car dealer had some magic crystal ball, and they said, “That's not the right car for this kid of yours, what he really needs is one from our special scratch and dent collection, and maybe one of these with a good tailwind and a nice downhill. It gets up to 65. It’s the perfect car for him,” I think I'd be pretty pissed off actually.
On the other hand, it could actually avoid some tragedy and is probably correct.
So how do we intervene in a way that empathizes with the consumer and yet actually gets them to where they want and need?
Well, in healthcare, that's exactly what I have to do a lot. I'm a physician.
This isn’t an unusual scenario. Say you have a 16-year-old boy who has abdominal pain, nausea, and vomiting. He comes into my emergency department and his parents want a CT scan. Why? Because they searched on Google, WebMD, or ChatGPT and it said he has appendicitis.
It sounds like I'm blaming tech, but otherwise, it's the mother-in-law or someone else who says he has appendicitis. Someone always does.
But what do the parents really want? Do they want a CT scan? Or do they actually just want the kid to feel better? Maybe that's what it is. Or maybe they feel really anxious that there's something wrong with their child, and they're actually trying to get rid of that anxious feeling they have and they just need reassurance.
So, in this case, what someone states they want, and what they really need, are very different. I think that's glaring in healthcare, which is why so much of the consumerized tech fails in healthcare. But I actually think that's true, like with our car example.
This is another scenario, and it's very similar to the one I had a few weeks ago. And if you haven't figured it out, I work in the emergency department. That's where I practice.
A 31-year-old woman comes in at 3 am on Friday with back pain. She wants an x-ray of her back. I find out it started four years ago. So why is she here at 3 am for an x-ray of her back? It hurts after work, and it’s better when she has a few days off. Okay, maybe there's some sort of exposure thing going on here.
She works as a waitress and she carries heavy trays, so the pain is probably from carrying heavy trays at work. My back hurts just thinking about that. That's hard work. And yet, she's here at 3 am. Why?
Well, it turns out her younger brother was recently diagnosed with cancer. She searched Google for the causes of back pain, and it's all cancer. So what does she want? Radiation? I'm guessing that's not it. A big medical bill? Well, whether she wants it or not, she's getting it. A note for work so she can do dinner with her brother the next night? Reassurance that her back pain isn't from cancer? Maybe that's what it is.
And then we get into the harder thing of, What does she need? Who thinks she needs an x-ray to diagnose her back pain? Not me. Who thinks that maybe an x-ray is a reassurance she needs to get on with her life and not have anxiety about this? Maybe. So should I get an x-ray of her back or not?
If I were to try and build a large language model or any sort of data model around this, where would I get the data to figure out that that's really what was going on and to actually deliver what I want to deliver?
Bridging healthcare and innovation
We’ll take a quick pause to talk about my relationship with healthcare and innovations. Where am I coming from?
I'm the VP at Inflect Health and Vituity. We staff about 450 hospitals, we're the largest physician partnership, and I oversee our innovation hub.
I still practice ER. I do about six shifts a month. I also have a Ph.D. in stats, so I have a lot of experience to bridge those worlds.
Since 1991, I've been building and deploying software, and I have a couple of patents. We’ve developed and deployed about 30 tools successfully, and we had about 300 that we were unsuccessful with, so it's about a 10% success rate, which isn’t great. In medicine, it wouldn't quite cut it. And now I advise companies large and small.
What this talk represents in these four things I'm trying to convey is that I have experience and observations that have led to many hypotheses. Every day, I'm reminded that I don't have any answers.
Identify the right technology for the problem
So, the first thing that we're talking about is that you have to understand the problem, and you have to understand what the real deep root of the problem is from many perspectives.
The second is that you need to understand what the technology is so that you can use the right technology. In this case, we're seeing that hard hats do in fact protect heads. But I don't know that they protect the head in certain situations.
One of the things that I think we all have to combat with the technology aspect of things is when we see cool tech like large language models or ChatGPT come out. There comes this halo effect of what you can do with this technology, and it fixes everything.
I remember when I first drove a Tesla back in 2013. It was my first experience with an electric car, and I did what I think most people do. You get in and you slam the accelerator down as hard as you can to see what happens, and you go, “This is amazing acceleration.” It was fun. It was a completely new car experience.
And then I started thinking about all the things that having an electric car and electric drive train can do for the car. And I was coming up with things like, It's a new car, it's a new experience, the seats are going to be more comfortable, the airbags are going to be better.
What performance characteristics around that electric motor are going to make the seats and the airbag better? It doesn't happen. And yet, with technology, when we apply it, there comes this halo effect where we start applying the wrong technologies.
And so that gets to the important aspect of matching the technology with the task. If we wanted to understand a prompt and respond based on training data, a large language model works pretty well. And it works better with more parameters and more training.
If we want to problem solve through hypothesis formulation and testing based on a conceptual model, which is a diagnostic challenge in medicine, then we probably need more traditional symbolic AI or a hybrid AI system.
If we're trying to prevent and predict an event, like having a heart attack based on individual data, now we're getting back to more typical ML models or a more definitive approach.
If we're trying to understand goals and context, mind a person to determine real needs and desires. The Theory of Mind problem, that's what I was talking about to some extent with the back pain example. The language model is excellent for communication with the person.
But how are we going to teach that language model to understand that that back pain was about concern for cancer if that's not made explicit? How do we train models where there’s no training data available?
We can think of online retail as another example, with users trying to resolve their frustrations with a purchase. Well, a language model may be a great way to provide empathic communication back to them. But if you're trying to figure out what you want to offer to assuage them, whether it's a return, refund, or gift certificate, an ML predictive model is probably better.
Most healthcare is figuring out what the patient actually wants and what they actually need. And that sounds really weird because we're used to the consumer aspect of things where you go from ‘want’ equals ‘need.’
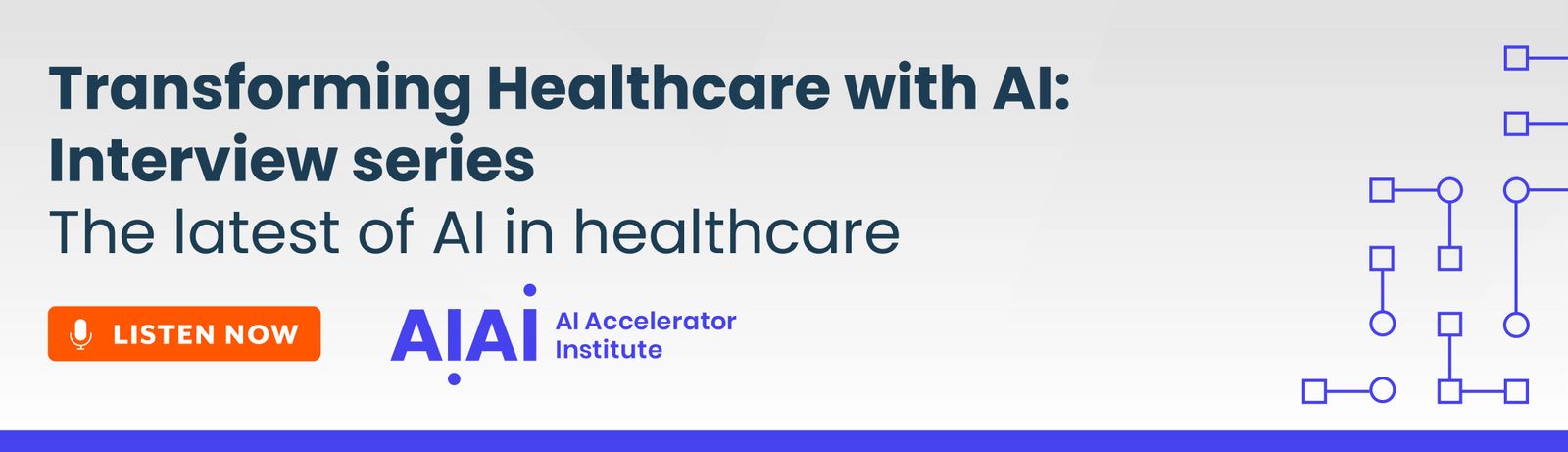
But if you actually think of your own healthcare experience or the healthcare experience of your loved ones and people you know, you go to the doctor and they say, “Oh, gee, I don't know what it is, I'm going to do all these tests.” And then they say, “I don't know, you're going to have to go see a specialist.
Then you go to a specialist and the specialist does the same thing. They may refer you to another specialist, or they’ll say, “We need to do these other tests.” And at the end of a very long cycle, they say, “This is the pill you need, and you'll be better. Or they say, ”Don't worry about it.”
And if you think of what that whole episode of care is, most of that entire episode is figuring out what you needed, which is actually the reverse of most of the consumer things we do, where you say that what you need is what you want, and most of what we do is now trying to fulfill that need.
What is the right technology for high emotional intelligence and compassionate problem-solving? If I had answers to this, I'd probably be retired.
Can a large language model that makes sophisticated next-word predictions formulate and test hypotheses? It's had other phenomena emerge that I wouldn't have predicted.
And how about something with a knowledge graph and predefined rules?
And of course, healthcare is also operations, logistics, communication, financial transactions, and monitoring. There's a business and operational side to it too.
Meet the needs of stakeholders, shareholders, and users
First, understand the problem being solved. We understand the problem and we understand the right technology. But I think of it in terms of users, shareholders, and stakeholders. Many of the implementations that I've had fail were because I ignored one of these groups.
If we think of our car buying example, the user was the kid driving the car. The shareholder is the one who's financially involved in the transaction, so that would’ve been the parents. And the stakeholders are everybody else affected by the kid driving that car, which are probably siblings, other drivers on the road, the insurance company, auto service maintenance, the garage shop, and the dealership.
One of the other places where I think this has been done wrong in tech is Google Glass. The user was the person wearing the glasses. The shareholder, the person making the purchase decision, was the person wearing the glasses. So that was nicely aligned and made that easy.
But then you had the stakeholders, which were the people sitting across from the person wearing the Google Glass who had different nomenclature for that person. So, you didn't get adoption. They didn’t align all three of those groups.
What I often find is that start-ups sell really cool technology to somebody like an insurance company or a hospital, but they don't align all three of the things. If you don't align with a stakeholder, no one's paying for it. If you don't align with the shareholders, you don't get the sales cycle and the traction takes too long. And if you don't align with the user, you never get value from that solution.
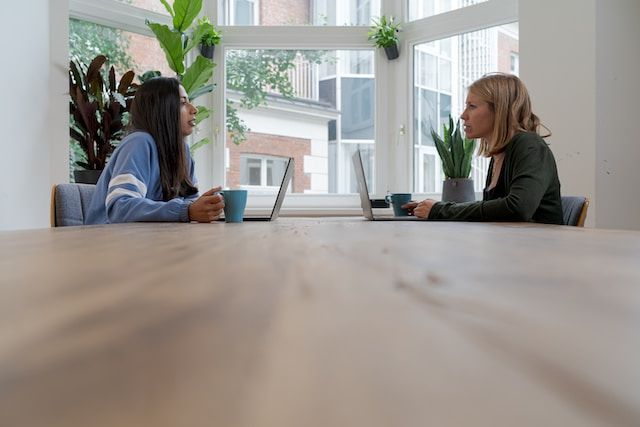
Invisible AI creates radical change
So now we’ve understood the problem, we’ve identified the right technology, and we’ve developed a solution that appeals to the user, the stakeholder, and the shareholders. But how do we get people to adopt the new technology?
About seven or eight years ago, we created a really cool ML model that predicted a patient's cardiac event in the next 30 days. The model fired at the time that they arrived at the emergency department. By any measure, it was an amazing model.
I said, “Okay, now we're going to implement our amazingness.” And we put it as a pop-up in the electronic health record. That didn't work. No luck there, it didn't change behavior at all.
I figured that I just wasn’t delivering this message correctly, so we implemented it as a separate app that ran on the desktop where the physician was working. But that didn’t do anything either.
Then we thought, Everyone loves text messages, right? We can be modern and do text messages. So the physician got a text message and it was secure. It was a pain to do, but that didn't work.
The physicians in this three-hospital system we were doing this in had a scribe working with them, so we notified the scribe to notify the physician. But no, that didn't work either.
If you're wondering what that looks like, unimplemented brilliance looks an awful lot like a cool patent plaque on the wall, and absolutely nothing more. Definitely not worth it.
How did we modify and change that for another iteration of it?
Another company that we were working with was looking into sepsis. Sepsis occurs once your body gets to the end of some infectious process. It’s the final common pathway for infection when your body's failing you.
So, who dies from sepsis? We looked at the data and we found that there are two groups, people who are super sick on arrival, which anyone can recognize. And you need better treatments for that. You don't need a pop-up, you don't need notification, none of that. You just need better treatments.
There was another group that actually wasn't septic on arrival, they just had an infection and looked kind of sick. But they then became septic later in their hospital state, 24, 48, and 72 hours later. So we said, “That's the group we need to identify and treat earlier.”
And so the company we were working with created an ML model to solve that specific problem. They identified the people who didn't know the signs of sepsis when they got there and became septic 24, 48, and 72 hours later.
We implemented it as a lab test this time. For the physician, it just looks like a lab test that comes back. They have no idea that there’s a sophisticated model running in the background that makes it easy for them to incorporate it into their workflow and makes it easy to know what to do with it because we know what to do with lab tests.
So, how do you implement AI? Well, if it's cool, that’s second place. If it's invisible, that's a radical change. When AI's invisible, it works great. But it can't always be invisible. Sometimes you have to have your user interact with it. So how can we do that? Here's my hypothesis of what we've been doing to get that done.
As human beings, we’re meaning makers, and we make meaning through narrative and story. And what that means is that we anthropomorphize like it's going out of style. So when we call something artificial intelligence, and we need mathematical equations and neural networks, we shouldn't be shocked that people anthropomorphize that.
And you have something like the gender of technology, where that communication seems and feels human. We know that it's not, but we attribute other human skills like reasoning, empathy, and intention all of a sudden to these things. And that's seductive, but it leads to using the wrong technology to solve the problem.
I feel like the future is built by the engineers who understand the technology and then destroyed by the pundits who misplace their hopes and dreams with technology that's not going to solve their problems.
So, what does that have to do with how we implement it when we can't get away from having the user needing to interact with the technology?
I believe that among the pundits and strategists, the strong human drive to anthropomorphize represents one of the greatest risks to realizing the real-world benefits of AI and advanced technologies.
But in the setting of implementation, I actually think it's a great opportunity to make that technology easy for people to adopt into their normal routines.
We have a cool AI system that does patient intake and diagnosis and everything. We call it a virtual medical resident. People know how to use it.
When my physicians ask, “How do I use ChatGPT?” I could describe it in a million ways. But if I say it's like a brilliant intern that's mildly intoxicated, that's exactly how you use it. You have to supervise what it does and you have to coach it. Every once in a while you're shocked by its brilliance, and every once in a while, you're shocked that it's drunk.
Another way we can think of that is through a smartphone. Why on earth is that called a phone? Because when it was rolled out, you knew what to do with it. It felt comfortable. If someone said, “Here's a computer that tracks your every movement that we're going to give you to carry in your pocket,” iPhones would probably not be the dominant player.
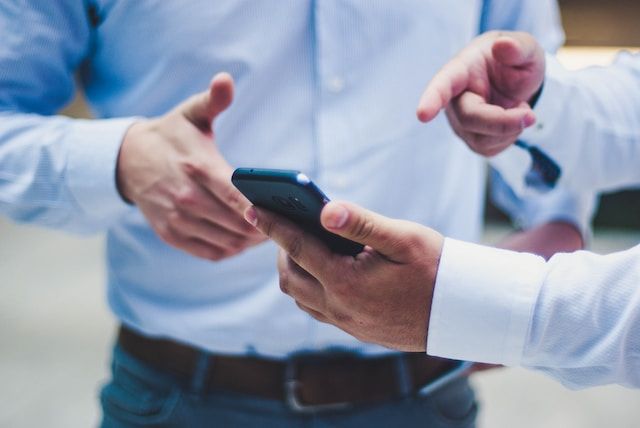
The importance of embracing hope
So, in summary:
- Deeply understand the problem.
- Understand the right technology for the right problem.
- Make the solution meet the needs of the stakeholder, the shareholder, and the user.
- Implementation is invisible, or it's highly relatable to an analog world.
My final plea to everybody is if you've been in the position where you implement, and I hope everyone is or will be because there's something magical about it, most of the time is spent in frustration. And that frustration, at least for me, can easily bleed into anger. But anger is an easy emotion, and I’d encourage you all to embrace the difficulty of hope, which is far more demanding of you.