“The future of marketing will be driven by artificial intelligence, and the companies that embrace it will win." – Marc Benioff, CEO, Salesforce
The marketing landscape is no longer a hazy realm of guesswork and intuition. In 2024, it's a data-driven battlefield where AI reigns supreme.
Forget the days of one-size-fits-all campaigns and scattershot strategies. Today, it's about hyper-personalization, predictive analytics, and lightning-fast campaign optimization – all powered by the brains of advanced artificial intelligence.
Think about it: 80% of consumers are more likely to do business with a company that offers personalized experiences. And with AI, that's no longer a pipe dream.
Imagine crafting individual customer journeys so tailored and so relevant that each interaction feels like a whispered secret just for them. Imagine predicting customer needs before they even surface, anticipating their desires like a seasoned magician. That's the magic of AI in marketing.
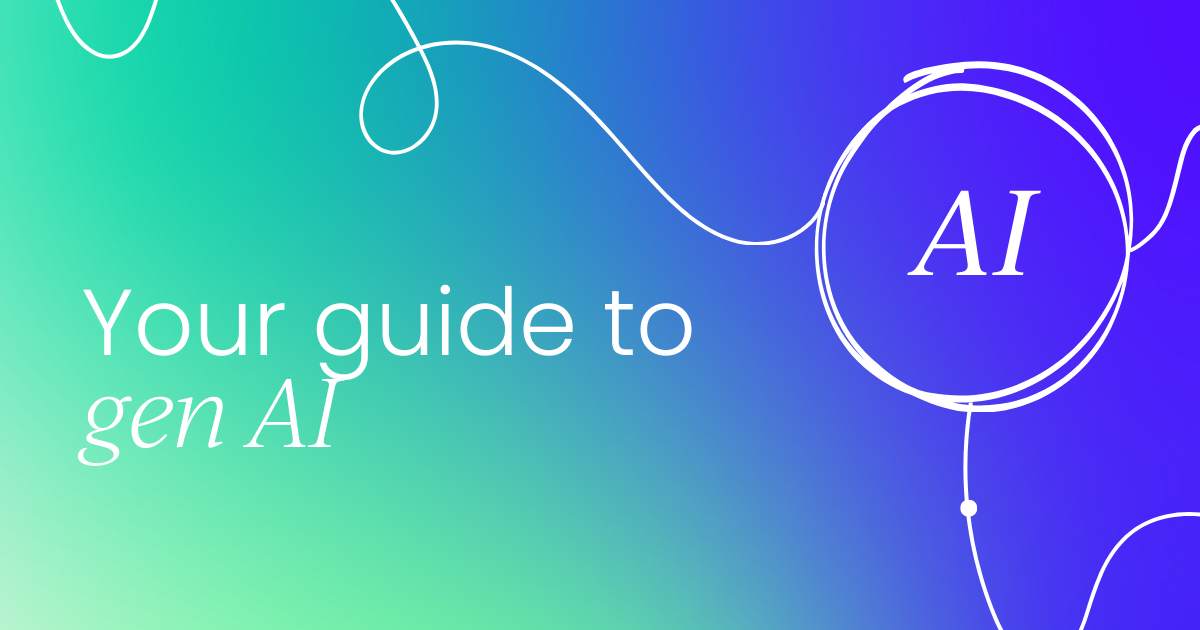
But wait, there's more! AI isn't just about mind-reading. It's about freeing human marketers from the shackles of tedious tasks and data crunching.
Imagine reallocating your time from A/B testing and campaign monitoring to crafting killer creative and building meaningful brand connections. AI automates the mundane, leaving you to unleash your human magic – the kind that builds trust, sparks emotion, and tells a story that resonates with your customers' souls.
But hold on, before we get swept away in the AI hype, let's address the elephant in the room: the challenges. Data privacy concerns, algorithmic bias, and the potential for job displacement are real. But here's the thing: AI isn't a monster to be feared. It's a powerful tool to be wielded with care, collaboration, and a deep understanding of human ethics.
This is where the future of marketing gets truly exciting. It's not about AI replacing humans, it's about humans and AI working together in a symphony of brilliance.
We call it collaborative intelligence, and it's the key to unlocking the true potential of AI in marketing. It's about using AI as a magnifying glass for human insights, a sounding board for creative ideas, and a tireless partner in the pursuit of marketing excellence.
This article delves into the transformative role of AI in advanced marketing and explores how collaborative intelligence can navigate the challenges that arise.
AI's superpowers for advanced marketing
Forget the marketing of yesterday. Today, AI is your secret weapon, a data-driven oracle that unlocks a new era of customer engagement. Here's how AI's superpowers elevate your game:
Predictive precision
Demand foresight
Analyze vast datasets of purchase history, social media trends, and economic indicators to predict future demand with 90% accuracy (source: McKinsey & Company). This means you can anticipate customer needs before they even whisper them, tailoring campaigns and products for maximum impact.
Micro-targeting magic
Go beyond demographics. AI identifies hidden patterns in customer behavior, predicting which products they'll love, what content they'll devour, and even when they're most likely to buy.
Imagine personalized ads that feel like mind-reading, emails that arrive at the perfect moment, and promotions that resonate with each customer's unique desires.
Trendspotter on autopilot
Forget chasing trends. AI identifies emerging cultural shifts and consumer preferences before they hit the mainstream, allowing you to ride the wave of innovation and stay ahead of the competition (source: Gartner).
Think trendsetting pop-up experiences, viral social media campaigns, and products that tap into the zeitgeist before anyone else.
Hyper-personalization
Customer chameleons
Craft individual customer journeys that feel like bespoke experiences. AI analyzes purchase history, website clicks, and social media interactions to create personalized content, recommendations, and offers that resonate on a deeply personal level.
Imagine dynamic landing pages that adapt to each visitor, emails that reference past purchases, and loyalty programs that reward individual preferences.
Emotional resonance engine
Go beyond just targeting interests. AI analyzes sentiment and emotional cues to understand how your customers feel about your brand, allowing you to tailor messaging and experiences that resonate on an emotional level.
Think heartwarming video ads, humorously targeted social media posts, and customer service interactions that feel like genuine conversations.
Dynamic optimization dynamo
Forget static campaigns. AI continuously monitors and adjusts your personalization efforts in real-time, ensuring that every interaction is perfectly timed and relevant.
Imagine A/B testing on hyperdrive, optimizing content, offers, and even website layouts based on individual customer behavior.
Automated optimization
Campaign efficiency unleashed
AI automates tedious tasks like budget allocation, channel selection, and ad bidding, freeing you to focus on the big picture. Imagine campaigns that optimize themselves, maximizing ROI and minimizing wasted effort.
Real-time insights radar
Forget waiting for data reports. AI provides real-time insights into campaign performance, allowing you to make instant adjustments and capitalize on opportunities as they arise. Think of it as a live dashboard that shows your campaign's pulse, letting you react with lightning speed.
Creative fuel injection
AI isn't just about automation. It can spark creative ideas, generate unique content formats, and even personalize your brand voice. Imagine AI-powered brainstorming sessions, personalized video ads, and social media posts that sound like your brand but speak directly to each customer.
Challenges and the rise of collaborative intelligence
While AI's marketing superpowers are undeniable, integrating them requires careful navigation. Three major challenges loom: data privacy, algorithmic bias, and job displacement. But fear not, for collaborative intelligence emerges as the guiding star, ensuring responsible and ethical AI implementation.
Data privacy
72% of consumers are concerned about how companies use their data (source: Pew Research Center). Marketers must prioritize transparency, ensuring clear consent mechanisms and robust data security protocols. Think layered data anonymization, granular permission controls, and readily accessible privacy policies.
GDPR and CCPA regulations raise the bar for data collection and usage. Marketers must comply with these evolving legal frameworks, building trust through ethical data practices and responsible AI deployment.
Algorithmic bias
AI algorithms can inherit human biases, perpetuating discrimination based on race, gender, or socioeconomic status. Marketers must actively audit their AI models, identifying and mitigating potential biases. Think of diverse training datasets, fairness-aware algorithms, and human oversight to prevent discriminatory outcomes.
Explainable AI plays a crucial role. Marketers need to understand how AI algorithms reach their conclusions, ensuring transparency and accountability. This allows for addressing biases at their root and fostering trust with customers.
Job displacement
AI automation may displace some marketing roles. However, it also creates new opportunities for higher-level strategic thinking, creative ideation, and data-driven decision-making.
Marketers must adapt and upskill, focusing on skills that complement AI, such as critical thinking, emotional intelligence, and human-centered design.
Reskilling and upskilling programs become essential. Companies must equip their marketing teams with the skills needed to thrive in an AI-powered future, fostering collaboration between humans and machines.
Collaborative intelligence
Human expertise remains irreplaceable. Marketers leverage their understanding of customer psychology, brand identity, and ethical considerations to guide AI, ensuring responsible implementation and alignment with brand values.
Continuous learning is key. The marketing landscape is dynamic, and AI models need to adapt. Marketers establish feedback loops where human insights continuously train and refine AI algorithms, ensuring their effectiveness in a changing world.
By embracing these collaborative intelligence principles, marketers can unlock the full potential of AI while mitigating its challenges. Remember, AI isn't a replacement, but a powerful partner in the quest for marketing excellence.
Conclusion
The future of advanced marketing lies in harnessing the power of AI while navigating its challenges through collaborative intelligence. By embracing a human-centered approach, building trust through transparency, and fostering continuous learning, marketers can leverage AI to unlock a new era of personalized, effective, and ethical marketing experiences.
In the AI era, the most successful marketers will be those who combine the power of machines with the magic of human touch.
Meet, connect, and network with peers at in-person meetups around the globe:
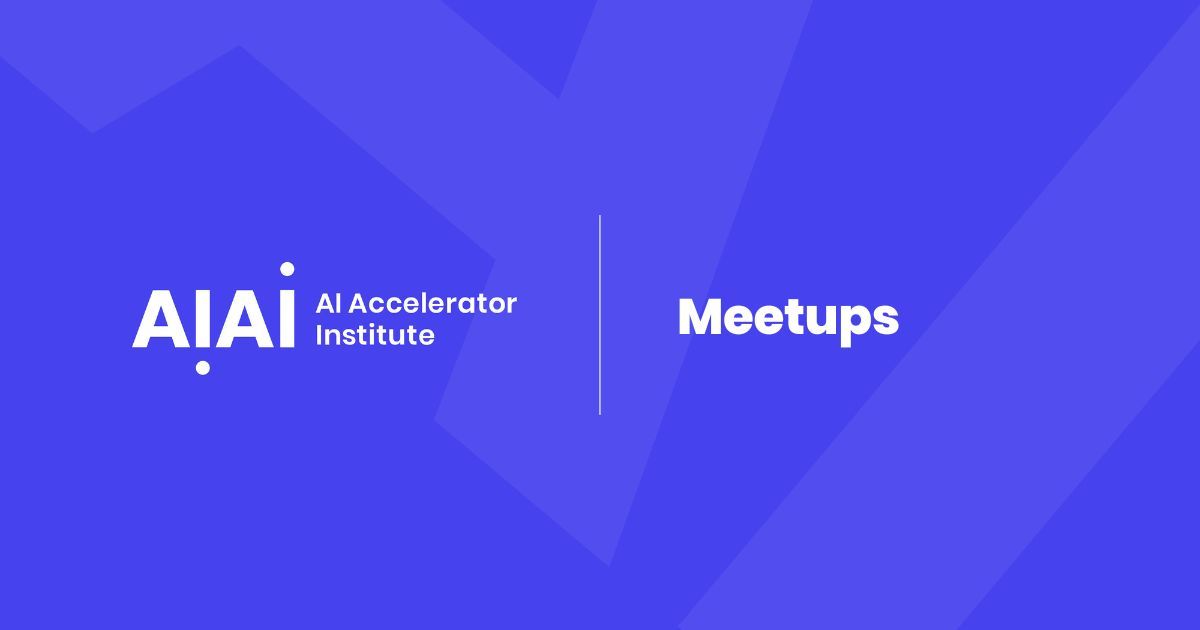