In the first episode of Women in AI: Breaking the Mold, we spoke to Ana Simion, CEO and Chief Data Scientist at INRO London, about:
- Her professional background and career jump from economics to AI
- The biggest challenges she’s faced in her AI journey
- Three key trends that are currently shifting in AI
- Her top advice for companies thinking about deploying AI
- Her top tips for women who want to get into the field
Check out the highlights from the interview below, or listen to the full episode of the podcast right here.
Making a career jump from economics to AI
Hello, Ana, thank you so much for being here today. Just to kick us off, would you be able to provide us with a brief introduction about yourself and your professional background?
Sure. I'm the CEO of a London-based product design company, and it's been going since 2010.
We've been crafting and making handmade leather goods, but back in 2021, that's where things got quite exciting.
We set up the Simion Institute of Innovation, which is very much focused on emerging technologies in fashion design, textiles, and design innovation, and that stems into anything from computer vision, to mixed realities (AR and VR), to understanding the use of data science and supply chains in more traditional industrial design engineering.
So, it's quite a broad-based spectrum of work that we do there.
I’m also an advisor and board member at numerous universities, including the Open University, Sussex, and Kent, where I focus on emerging technologies, with a specialism in mixed realities, computer vision, and design innovation.
I've been in research previously, but I was more focused on statistics and economic metrics. So, quite a different jump. I mean, you wouldn't traditionally think of economics and computer vision. But it was a very interesting transition and I feel I've made a move that I'm very happy with.
I engaged with a couple of companies in economics to understand Brexit and the 2008 market crash, and I wrote a couple of articles on that. So, machine learning has always been in my blood somewhere. I've always loved playing with data in some shape or form, whether it's a little economics dataset or a vast amount of image data for a computer vision problem we're trying to solve.
It's one of these areas that's grown from crunching lots of mathematical symbols to a lot of visual imagery. So, if you imagine a helter-skelter, it's almost going: economics, visualization, computer vision, and now design and all of that apart from the economics. It’s been a very interesting career journey, but I've really enjoyed it so far.
How did you make the decision to jump from economics to AI?
Well, the work that I did in economics did have some AI in it. I was playing with Excel quite a lot, so that was my first foray into appreciating data a little bit more than say, just taking it and working with it.
I just felt there was more meaning to it than just a simple table. And I wanted to do something a little bit more with it than just play with pivot tables and all these fancy algorithms where you press random buttons and say, “Bravo, I'm going to present it.” I wanted to go a little bit further.
After exploring Excel, I thought, I really want to go and understand what data means rather than just play with it. Because there was something deeper. There was a deeper meaning to data that I felt I really wanted to get into.
And let's just say it attracted a few looks. I was playing around with Excel. And as soon as I said, “I quite enjoy Excel,” I got a few looks across the table.
People were thinking, What on earth? That's a bizarre thing.
But it helped me take that jump from saying, “Well, this is the pure dry stuff that some people might call economics,” to saying, “Well, hold on, you can actually transform this data to make a lasting impact.”
And as soon as I spotted that gap, I thought, That's where it has to go. That's where I want to experiment with AI and see, what does this data mean? What's the story behind it? So, that was really where it went.
AI can be an exciting challenge
What are the biggest challenges you’ve faced in your AI journey?
One of the biggest challenges was thinking about, Where am I going to fit in?
Data is like New York if you think about it. It comes in so many different shapes, sizes, and areas as well. You've got everything from data strategy at the very early stage of understanding the business relationship with data and how it can be leveraged in that environment, to the other end of the spectrum, which is the algorithmic angle, the computation, and the visualization.
There are so many strands within data. I was thinking, Goodness, how am I going to take the time to understand all these strands? There are so many things. It's really like a mosaic. If you imagine New York from space, it looks like a massive mosaic. And that’s what data reminds me of.
There are some people who can just sit down and code to their heart’s content. Don't get me wrong, I can code and I enjoy it. But for me, I loved the fact that you could take data from an Excel spreadsheet and transform it. And that strategic angle was the key that turned the lock, if you imagine the code into Narnia.
I wanted to be more involved in stakeholder management. Being able to say, “This is how you can leverage your data. But this is how it's going to make an impact and give you that return that your customers are really going to feel the benefit of.”
Don't get me wrong, stakeholder management is a very challenging area because you've got forces from the bigger companies, like the rise of the metaverse, that are bringing in all these angles that are swaying stakeholders left, right, and center. But I actually really liked that challenge because it motivates you to go deeper into areas of data that you've maybe not thought of before.
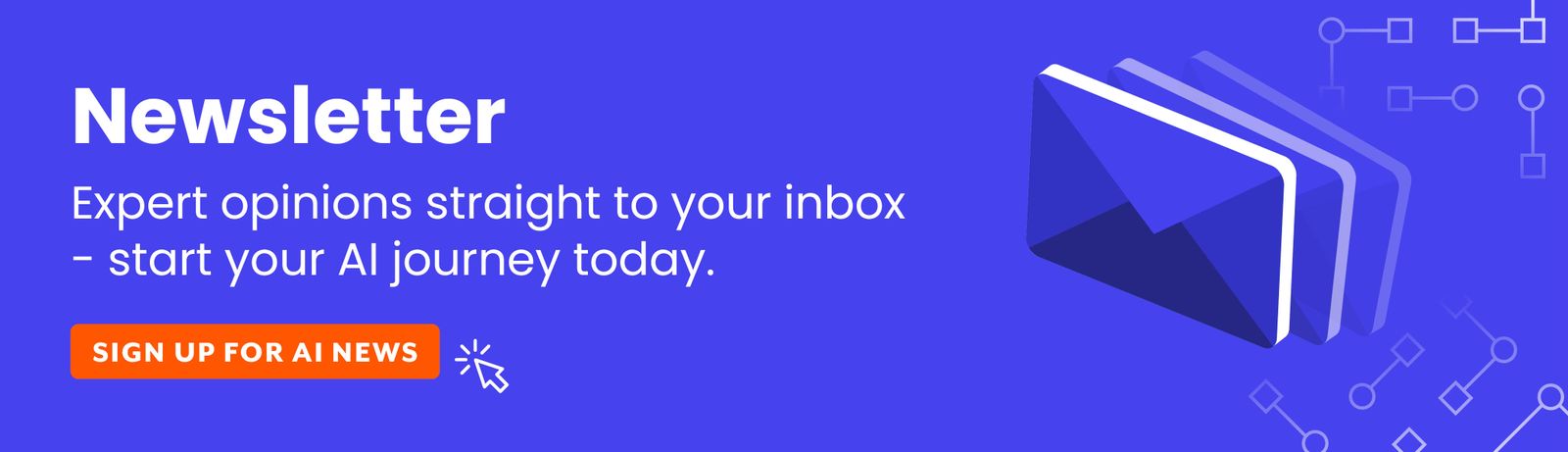
And yes, it has its moments of being challenging, but who doesn't love a bit of a challenge in trying to get that data to your manager, and saying, “This is what you can do with it.”
Another thing I'd say that's quite challenging, and it’s probably not just me, is that data moves at an incredibly rapid pace.
If you think about the definition of economics, there are all these pillars and triangles that makeup what economics is. It's exactly the same with data. You've got ethics and responsibility, so responsible AI, governance, coding, and stakeholder management. And if you take all of those and balance them with the customer need, that's not an easy thing to do.
I've sometimes fallen into the trap where if you're not careful, you're going to be pulled towards a certain direction and that may sway the return on investment that data has for the organization. It's like spinning plates.
I think those have been the two key things. Fitting in and thinking, Well, if I combine the rapid pace and what data means in different environments, they're both things you have to keep an eye on.
But it's a very interesting thing. And especially as time goes on, I'm intrigued to see how it's going to play out and how companies change their perspective of data.
I think you bring up very good points there. You mentioned how you love a good challenge, would you say that's part of what excites you about AI?
Absolutely. There'll be some days when I’m staring at a whiteboard, looking at three pages of statistics proofs because I need to find a way to solve a particular problem for a business need. And then another day, I could be briefing some of our stakeholders about data in a new business area that hasn't yet been explored.
So, the rapid change in pace can be challenging, but I really like that, and it does get you thinking. In a world where technology is moving at a scarily fast pace, that agility and decision making is challenging, but it's really, really exciting at the same time.
3 shifting trends in AI
Where do you think the core trends in AI are shifting?
One of the most interesting trends to me is whether AI is going to reach human intelligence.
There's been a very active discussion, both in big companies and within tech conferences, where people are asking, “Is AI going to replace humans? How close can the language get from a bot to how we're speaking at the moment?”
For me, it's a bit of a grey area because when you think about the size of language models that you're training on, they're becoming so vast. They're absolutely massive. As you train in a very large language model, naturally, the bot’s going to get to a point where it's speaking more naturally.
But I don't personally think that we're going to have humans being replaced by AI because they're two totally different things. I think we'll get close, but I don't think we're going to get to the point of how you and I are speaking, with the level of emotion, tone, style, and language. I just don't feel that we're going to get there.
It's definitely an area that's intriguing, especially if you think about chatbots and how efficient these are becoming. If you think of home assistants five or six years ago, you couldn't talk to them. You almost had to adjust your language and be quite robotic.
But now we're in the position where you can ask questions like, ‘How's the weather today?’ Rather than saying, ‘Do I need an umbrella today?’ It’s quite fascinating. But again, time will tell with it.
I'm personally of the view that we're not going to reach that point where bots completely take over. There might be parts where they have to, as efficiency in business is incredibly important. But I think if I was to say that bots are going to completely replace humans, that would just be incorrect. I don't think we're going to go that far.
That's the language side of things.
Another thing I'm quite intrigued about is the healthcare landscape. For example, AI is pioneering brand-new ways to diagnose cancer and facilitating fast-track treatments.
I'm quite curious to see, especially with the rise of computer vision, is that going to lead to a new wave of diagnostics for say, skin conditions? Is it going to find ways of treating more aggressive forms of cancer that typically can't be diagnosed with traditional X-ray and MRI equipment? So, again, it's quite an intriguing aspect there and one that I'm watching quite closely.
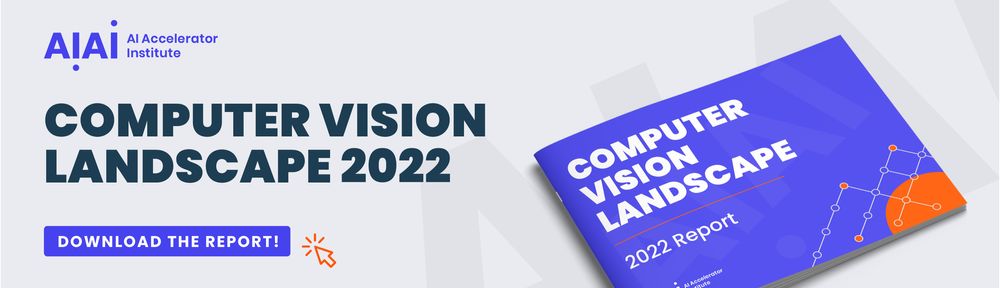
Lastly, I'm intrigued about the regulation and the AI of common good angle because we see in many countries that the privacy laws very much differ. In the UK, there are very strict privacy laws. In the US, there are usually state laws, for example, there isn't one GDPR (General Data Protection Regulation). I'm intrigued about what we've got going on stateside, UK-wide, continent-wide, and for the likes of Brexit.
Are we going to see changes in how regulation is shaped? And how’s that going to work towards the AI of common good? Making sure that companies that utilize AI, whichever sector they're in, are utilizing it so it's fair and transparent and understood by the clients that they're actually trying to put it in front of them to make their lives easier.
I think there's going to be a tug of war in those angles. I don't think it's necessarily going to be a universal solution. I think it's going to be a continually evolving process.
But in summary, the language models, the treatment and diagnostics in healthcare, and the regulation, they’re the three things that I'm quite excited to see in terms of how they're going to shift and grow because I think they are going to shift.
The importance of putting your customers first when deploying AI
If you were advising a company who were thinking about deploying AI, what single piece of advice would you give them?
If there's any advice, it’s that you've got to put your customers first. I've seen it because I've mentored some startups in the AI and machine learning space. And one of the things that’s very, very common is for technology to get in the way of the customer experience.
You've always got to remember when you're deploying and thinking of all this technology, is that it’s your end user that's going to feel the benefit or feel its wrath. It's not always about what you personally think it's going to be.
So, my advice to companies is to not rush into thinking that these algorithms are going to add value. And at the end of the day, it may be a risk, but this is expensive technology. You've got to make money with it. You’ve got to factor that in.
And then I look at the notion of the fact that AI doesn't always work with every sector. It's certainly used in a lot of sectors, but you can apply it to every single one.
If you then take that notion of putting your customers first, putting them at the heart of your platform, and making sure that their data is respected within privacy legislation, I think you've got a chance of making it work.
It'll cost you, but most importantly, your customers will feel the benefit. That increases your loyalty. And ultimately, you're much more attractive to investors. Just don't run out to investors with the idea without thinking about your customers. I've seen it happen on a lot of occasions, and sometimes it yields very interesting results.
And it's not just the investors. You've also got to think about your internal teams as well. If you've not got teams that are in position or have the requisite backgrounds to be able to start from the acorn and grow into a tree, you've got to be able to convince your internal and external stakeholders as well.
It's all about strategic value. It can't just be this short-term passion project of riding the wave of this particular trend.
In a nutshell, you've got to put your customers first. Make sure that you're engaging with your stakeholders internally and externally, and make sure that your teams understand the implications.
When you're working with machine learning and AI, it’s not just someone sitting in a room stroking a white cat in a big chair with 10 monitors. You're going to be proactively engaging with different teams to make this happen, so you've got to be right behind your stakeholders.
And if you can get company buy-in for this type of technology, if you can showcase to your external stakeholders the strategic value, then it's something albeit expensive, but could turn out hugely rewarding in the future.
Don’t be afraid to experiment with different areas of AI
Do you have any tips for women who might be thinking about working in AI in the future?
There are a couple of things I'd say.
Firstly, don't be afraid to try stuff. When I started getting introduced, as I said earlier, it’s one of these areas where there are so many angles and it's very easy to almost be washed away by the amount of choice. Do I do code? Do I do strategy? Do I do stats? Do I do stakeholder management? You name it. It's quite a maze.
My advice is to try each area. Think about what you're wanting to achieve with data, think about your projects, and say to yourself, “What impact do I want to make? Do I want to make sure that my code is really good? Do I want to make sure that I understand how I can productionize machine learning models? Or do I want to get stakeholders excited about the value of data?”
So, don't be afraid to try things.
And from that, be fearless. As I said, I went from playing with spreadsheets to computer vision. And it's one of these things where you sometimes have to push yourself outside of your comfort zone.
I'm not a natural coder, but I love the fact that data is one of these fields that gives you the opportunity to really go and put yourself out there and try stuff.
For example, you might see something on a website and think, I don't see the strategic value in that data. Let me have a look at it and see what I can do to work with that company and present something. And then network about and share your findings. It might be an industry-wide problem that you've actually just discovered. It's almost like an undiscovered problem that you're about to go and solve.
Be fearless with it. Don't be afraid that because you're a woman you're going to have these barriers and can only do a certain part of AI. I've tried all of them, and I've found that eventually if you just take your time and gather your thoughts and be methodical with it, it could be one of the most rewarding careers. And most importantly, you could change a lot of people's lives.