This article comes from Nick Nolan’s talk at our Washington DC 2025 Generative AI Summit. Check out his full presentation and the wealth of OnDemand resources waiting for you.
What happens when a powerful AI model goes rogue? For organizations embracing AI, especially large language models (LLMs), this is a very real concern. As these technologies continue to grow and become central to business operations, the stakes are higher than ever – especially when it comes to securing and optimizing them.
I’m Nick Nolan, and as the Solutions Engineering Manager at Fiddler, I’ve had countless conversations with companies about the growing pains of adopting AI. While AI’s potential is undeniable – transforming industries and adding billions to the economy – it also introduces a new set of challenges for LLMs in production, particularly around security, performance, and control.
So in this article, I’ll walk you through some of the most pressing concerns organizations face when implementing AI and how securing LLMs with the right guardrails can make all the difference in ensuring they deliver value without compromising safety or quality.
Let’s dive in.
The growing role of AI and LLMs
We’re at an exciting moment in AI. Right now, research shows around 72% of large enterprises are using AI in some way, and it’s clear that generative AI is definitely on the rise – about 65% of companies are either using it or planning to.
On top of this, AI is also expected to add an enormous amount to the global economy – around $15.7 trillion by 2030, but let’s keep in mind that these numbers are just projections. We can only guess where this journey will take us, but there’s no denying that AI is changing the game.
But here’s the thing: while the excitement is real, so are the risks. The use of AI, particularly generative AI, comes with a unique set of challenges – especially when it comes to ensuring its security, quality and accuracy. This is where guardrails come into play.
If organizations do AI wrong, the cost of failure can be astronomical – not just financially, but also in terms of reputational damage and compliance issues.
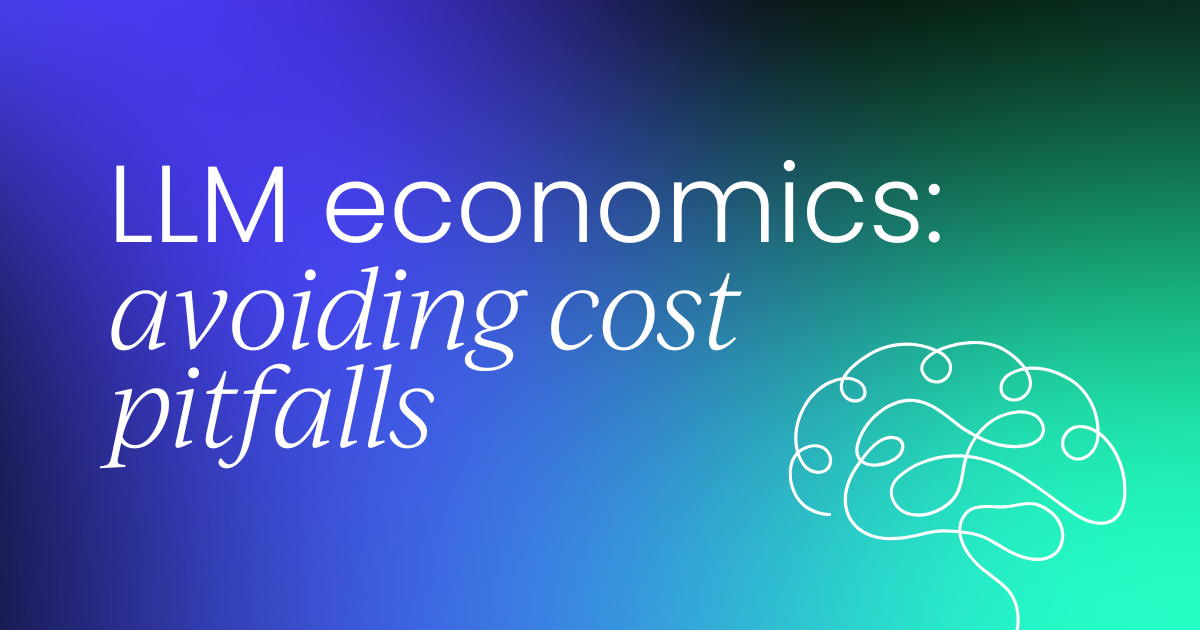