March’s news roundup saw AI (artificial intelligence) beating world champions at bridge, deep learning helping Google design smaller chips, AI spotting type 2 diabetes sooner, and more. The month of April was just as exciting, with further developments announced in the world of artificial intelligence.
From machine learning being used to predict natural catastrophes like earthquakes and volcano eruptions to AI giving Hawaii a helping hand in protecting its biodiversity, we’ve highlighted a few noteworthy studies.
In this article article, we look into:
- Deep learning predicting humans’ face judgments
- AI helping to protect Hawaii’s biodiversity
- Machine learning informing TBI outcomes
- How deep learning helps to detect earthquakes in noisy cities
- Machine learning seeing into volcanoes’ eruptions
- Machine learning predicting noise impairing hearing
Take a look at March's news round-up below:
Using deep learning to predict users' superficial judgments of human faces
Based on neural networks, researchers from Researchers at Princeton University and the Booth School of Business of the University of Chicago have tried to predict a few of humans’ automatic inferences when interacting with a new person, based solely on their faces.
The paper was published in Proceedings of the National Academy of Sciences (PNAS), introducing a machine learning model that is able to predict arbitrary judgments that users make about specific photos of faces - all with extreme accuracy.
One of the researchers, Joshua Peterson, and his colleagues noticed that, from previous studies focused on face judgments, only a few explored the topic through state-of-the-art machine learning tools.
Work from the team has resulted in the creation of an extensive and detailed dataset of face-related biases and stereotypes.
AI is helping to protect biodiversity in Hawaii
The Nature Conservancy (TNC) is using artificial intelligence and machine learning to find and track Himalayan ginger more efficiently. The weed was introduced in the 1950s as an ornamental and has since spread from backyards to rainforests. Himalayan ginger forms a dense mat on the ground, which doesn’t allow for other plants to be cultivated.
Hydrologic studies have found that it also uses up a lot of water. It controls the ground and changes how the watershed functions, not letting moisture or rainwater sink into the ground. This leads to erosion and water runoff into nearshore reef ecosystems.
Artificial intelligence can help find the weed much faster by speeding up the analysis, as it can be trained to identify the weeds in images and accelerate workflow. AI can be a great tool in helping the “endangered species capital of the world” preserve the health of mauka to makai and conserve what’s left.
Prognostic model uses brain scans and machine learning to inform outcomes in TBI patients
Developed by data scientists and neurotrauma surgeons from the University of Pittsburgh School of Medicine, the prognostic model is the world's first in using machine learning and automated brain scans to inform outcomes in patients who have traumatic brain injuries (TBI).
The team showcased their study in the journal Radiology, demonstrating an advanced machine learning algorithm that is capable of analyzing brain scans and relevant clinical data from TBI patients to accurately and quickly predict both the survival and recovery six months after the traumatic injury.
The custom artificial intelligence model processes various brain scans from each patient, combining them with an estimate of the coma severity and information from blood tests, vital signs, and heart function. Data irregularity was also accounted for, with the model being trained on different image-taking protocols.
It accurately predicted the risk of death and unfavorable outcomes at six months after the traumatic injury in patients.
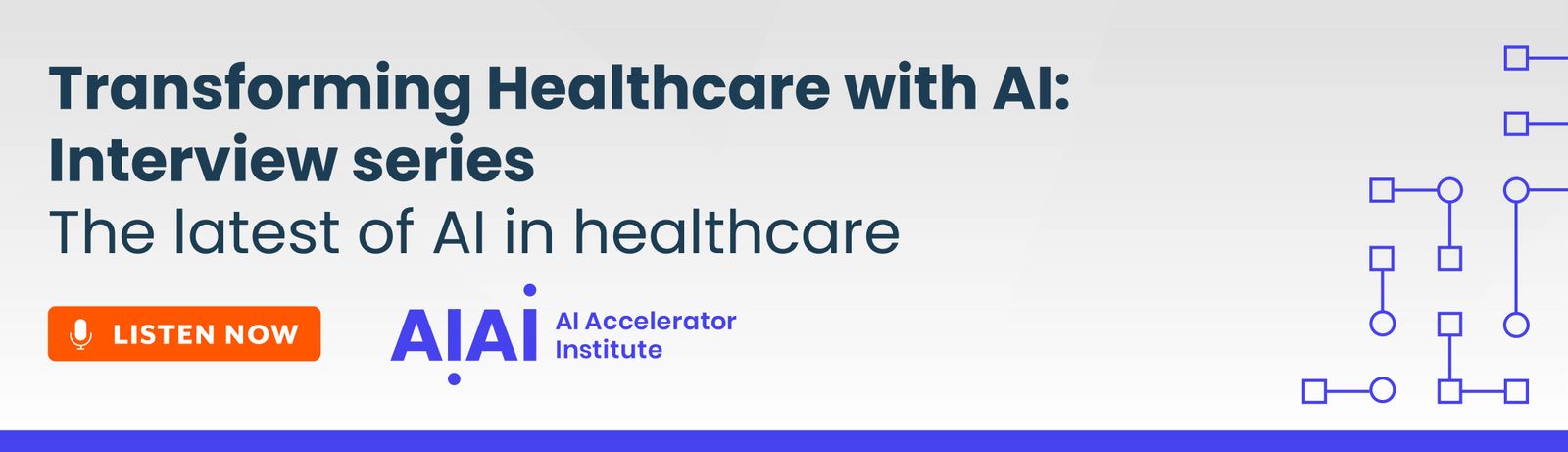
A deep-learning algorithm could detect earthquakes by filtering out city noise
In a paper published in Science Advances, researchers from Stanford declared they could improve earthquake detection and monitoring in cities and other built-up areas. Algorithms trained to sift out background noise could help in extremely busy and earthquake-prone cities in countries like Japan.
The team’s deep learning algorithm, UrbanDenoiser, was trained on datasets of 80,000 samples of urban seismic noise and 33,752 samples indicating the activity of earthquakes, respectively collected from Long Beach and San Jacinto, California.
When the algorithms were applied to datasets in the Long Beach area, they detected a lot more earthquakes and made it simpler to determine where they started. Datasets from a 2014 earthquake in La Habra, California, resulted in the team observing 4x more seismic detections in the denoised data, compared to the officially recorded number.
Machine learning helps see into a volcano’s depths
A study by Boschetty et al. (2022), Insights Into Magma Storage Beneath a Frequently Erupting Arc Volcano (Villarrica, Chile) From Unsupervised Machine Learning Analysis of Mineral Compositions, successfully applied an unsupervised machine learning technique to analyze the crystal cargo of various eruptions from a single volcano, overtime.
The technique, hierarchical clustering, allowed for the detection of crystal composition populations in multidimensional space that wasn’t previously obvious in other visualization methods. By combining thermodynamic modeling of magmatic fractionation with the results, researchers can then identify likely conditions for the formation of each cluster of mineral compositions.
As some volcanoes tend to erupt frequently while changing from explosive to effusive, this technique can help to map out the volcano’s plumbing system over time and aid in distinguishing what the products are of both explosive and non-explosive eruptions.
Machine learning predicts when background noise impairs hearing
Jana Roßbach and colleagues at Carl von Ossietzky University in Germany can accurately predict when people with different levels of hearing impairment and people with no hearing impairments would mishear more than 50% of words in different noisy environments.
The team used a speech recognition model, which is based on deep machine learning and uses multiple layers to extract higher-level features from raw input data. The model, when combined with conventional amplitude-enhancing algorithms, could extract phonemes, or units of sound that form words’ building blocks.
The algorithm was trained with recordings of random basic sentences from ten female and ten male speakers. The speech was then masked with eight possible noise signals, to create a simple constant noise, and another person talking over the speaker. The recordings were also degraded to different levels to copy how they would sound to people with various levels of hearing impairment.
The team then asked participants with various levels of hearing impairments and with no hearing impairments to listen to the recording and jot down the words they heard to determine the noise threshold causing listeners to mishear over 50% of words. The responses very closely matched the machine learning model predictions, with just a 2 dB error.
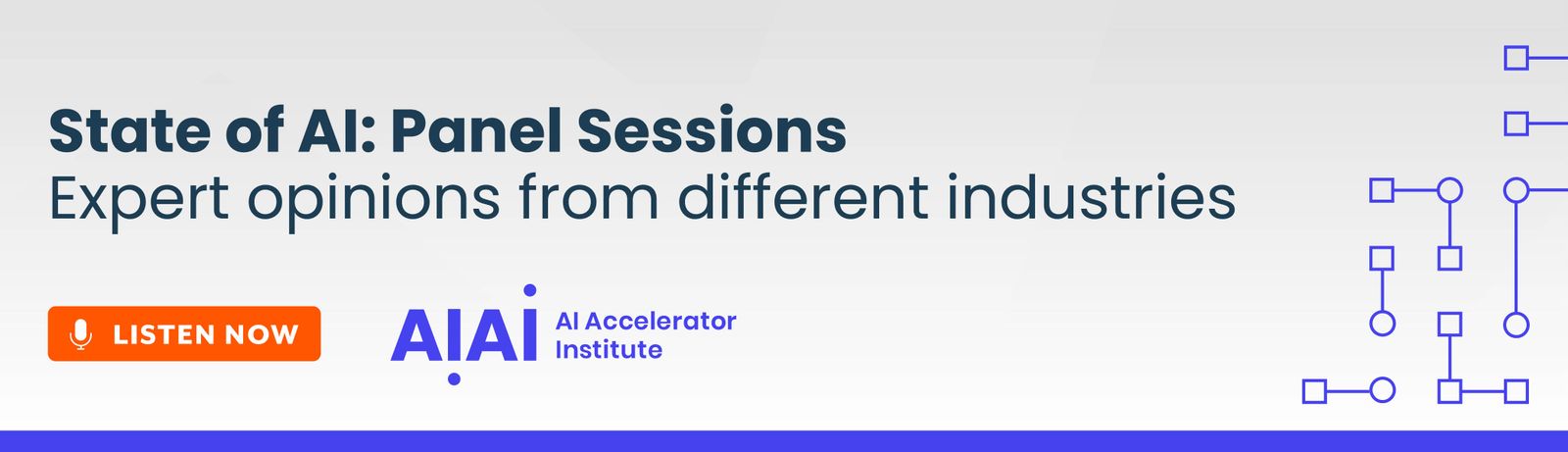