This time, we’re taking a look at what happened in the world of artificial intelligence (AI) in the month of June. In May, we saw how AI is helping to monitor coral reef health and even how automated blood devices are being developed to help lessen the burden in hospitals and other medical settings.
June keeps highlighting the importance of AI in medicine, while also giving us insight into early human behavior.
In this article, we'll take a look at a few headlines, including:
- AI helps Instagram to verify your age with your face.
- How AI is advancing structural proteomics.
- Deep learning framework identifies regions of COVID-19 infection in lung scans.
- Machine learning can be a successful tool to predict oral cancer risk.
- Scientists use AI to predict when early humans started using fire.
Find out more about machine learning below:
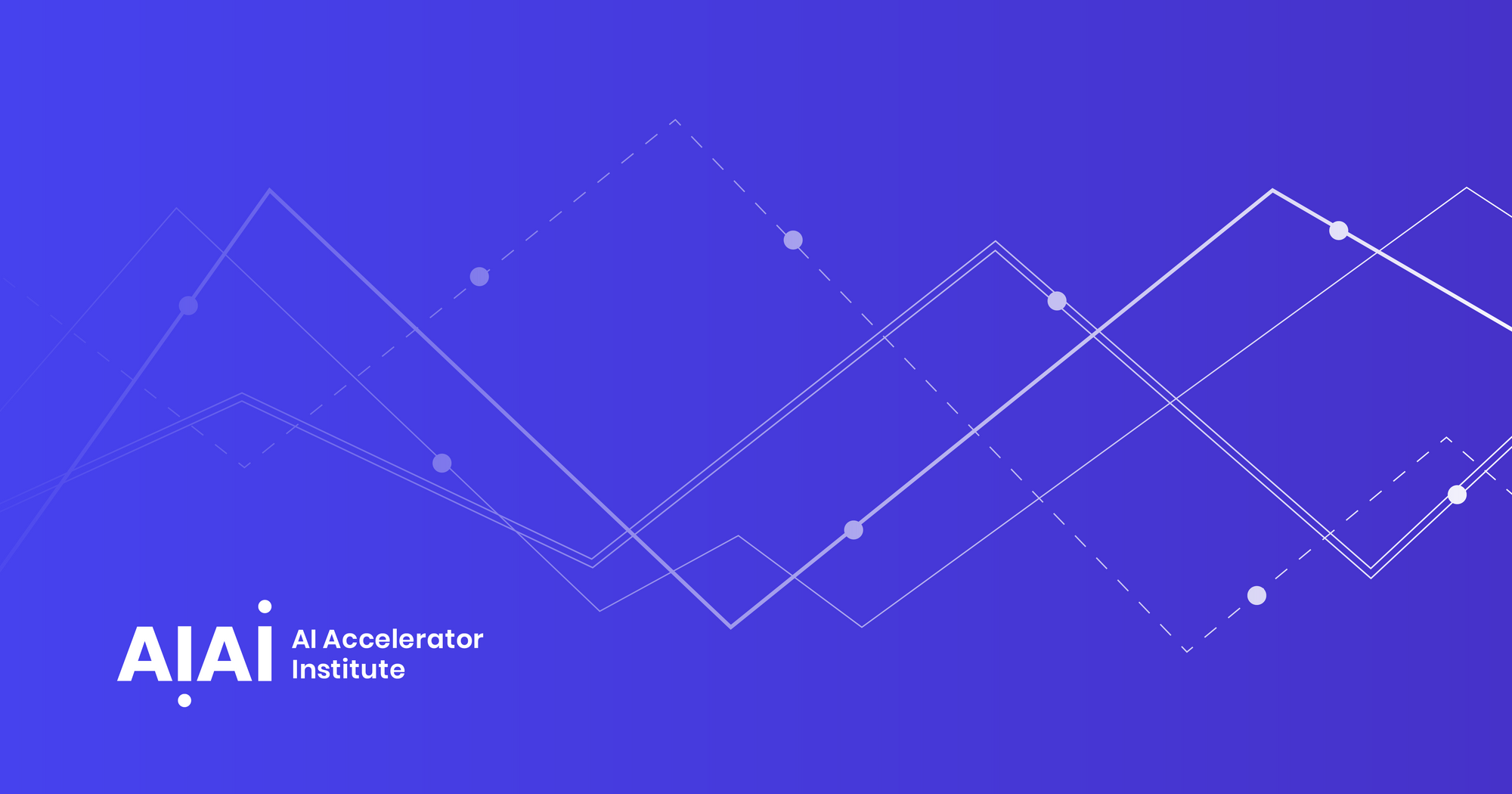
AI helps Instagram to verify your age with your face
The social media platform Instagram is testing a new age verification method that uses AI to estimate how old users are, via a video selfie. At the moment, users can send in photos of several types of ID cards, with US users having the additional options of social vouching and AI estimation.
Social vouching involves users selecting three people aged 18 or older who follow them, who have to confirm that user’s age within three days if Instagram requests it. The platform is also checking user posts for additional verification; if someone claims to be 21 but posts about their 16th birthday, Instagram will flag it.
How artificial intelligence is advancing structural proteomics
This article is based on yet-to-be peer-reviewed findings, and all results should be regarded as preliminary and interpreted as such.
Protein complex formation is essential for drug design and antibody development, however, proteins can attach to each other in millions of different combinations. Current docking solutions for predicting these combinations can be extremely slow, so faster and more accurate solutions are in demand.
A new machine learning model, EquiDock, was introduced in a pre-print published earlier in the year. It can quickly predict how two proteins will interact with each other, and the model doesn’t heavily rely on candidate sampling. It reached predictions up to 80-500 times faster than popular docking software.
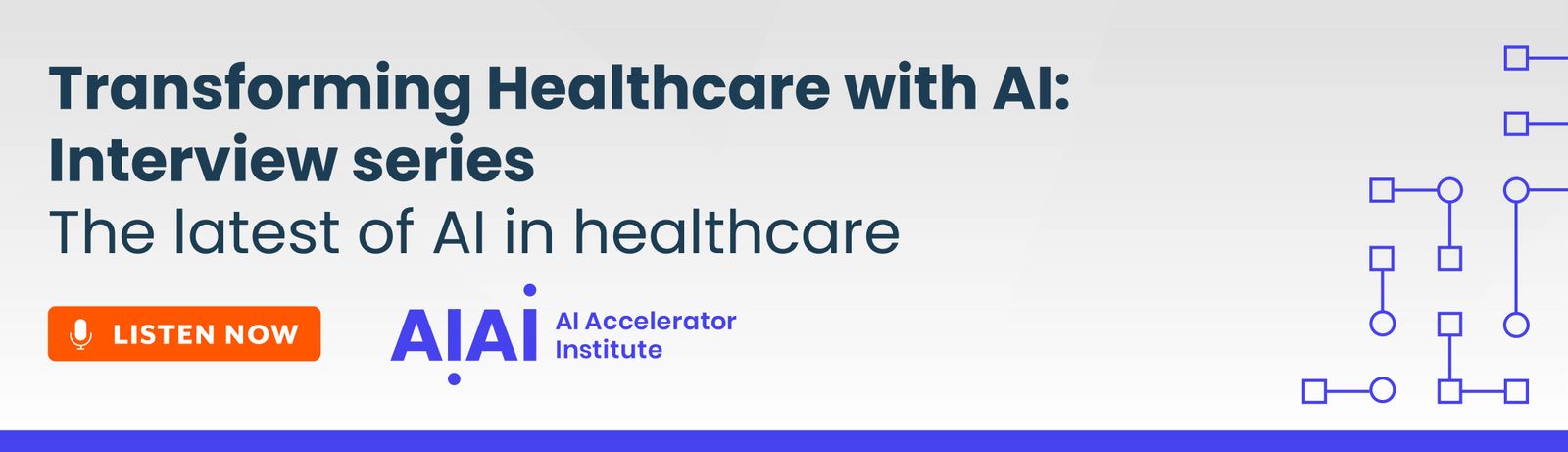
A deep learning framework identifies regions of COVID-19 infection in lung scans
Researchers from the UK and China have developed an artificial neural network that can identify regions of COVID-19 lung infection in CT scans. Interestingly, the network was trained with images from healthy patients.
The study proposes a new weakly supervised deep learning framework, which has been dubbed the Weak Variational Autoencoder for Localisation and Enhancement (WVALE). The framework has a neural network model called WVAE that uses a gradient-based approach for anomaly localization.
It works by transforming and recovering original data to learn about latent features, which makes it possible to identify anomalous regions. This approach is “weakly supervised” because only healthy control images were used to train the model and not a mix of CT scans from both healthy and COVID-19 infected patients.
The WVAE model can produce high-quality attention maps from CT scans, with fine borders around the infected lung regions. It also produces segmentation results that are comparable to those produced by a variety of conventional, supervised segmentation models, outperforming several existing weakly supervised anomaly localization methods.
Machine learning can be a successful tool to predict oral cancer risk
The study from John Adeoye of the University of Hong Kong, SAR China, was presented during the "e-Oral Health Network I" session, as a talk titled "Predicting Oral Cancer Risk using Machine Learning".
Aiming to develop a machine learning-based platform that predicts the risk of oral cancer and Oral Potentially Malignant Disorders (OPMDs), the study included visual oral examinations of 1,467 participants of a community-based screening program undertaken by three calibrated dentists. Each participant’s status was defined as positive/negative for oral cancer or OPMDs.
Those screened negative were monitored through state-linked electronic health records, with information on demography and other risks obtained. Histologic diagnoses and input features (n=40) were used to populate 12 machine learning algorithms, with an 80:20 train-test splitting randomly applied to the data during development.
Using recursive feature elimination with 10-fold cross-validation allowed for feature selection, and synthetic-minority-oversampling-technique with edited-nearest-neighbors was implemented for class imbalance correction. Internal validation was conducted with the unused 20% of data with the comparison of outputs by using McNemar’s test for optimal model selection performance metrics like specificity, recall, and F1-score.
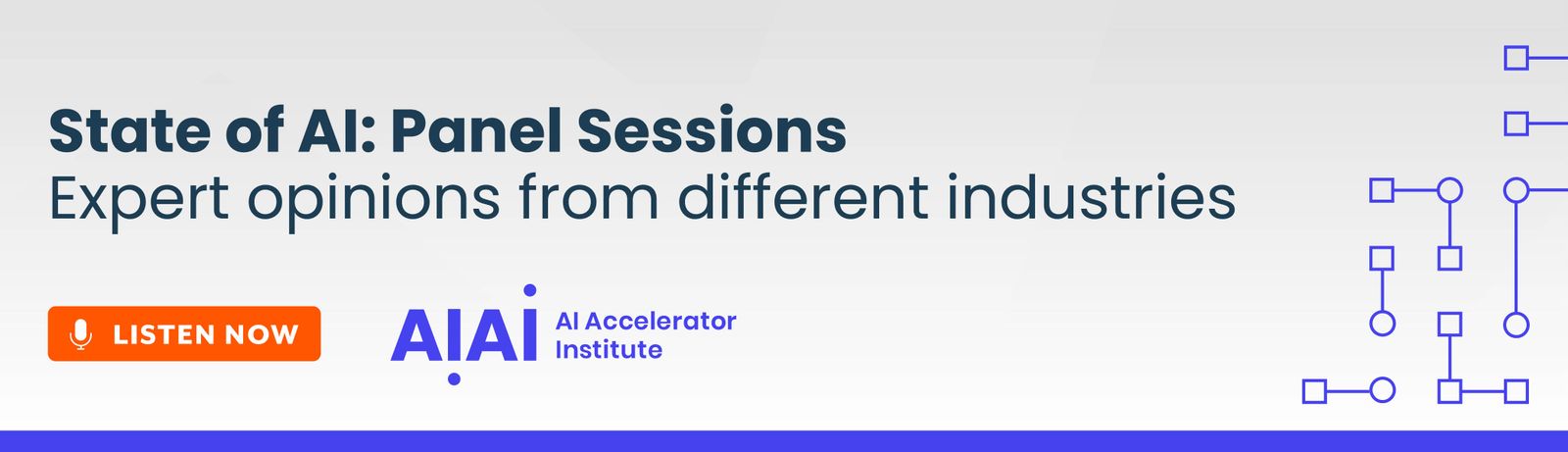
Scientists use AI to know when early humans started using fire
Researchers from the Weizmann Institute of Science published a study in the “Proceedings of the National Academy of Sciences”, where they may have found the remnants of an 800,000-year-old fire in Israel.
Experts used to need straightforward evidence like charcoal remnants to know whether there had been a fire, but such evidence can be difficult to find in ancient sites. Artifacts like stone tools, however, which were often made from flint, typically survive.
Researchers heated pieces of flint to different temperatures using spectroscopy (a technique that measures light absorption) to try to determine if the flint had been burned. The result? Extremely complex data, which led to the team developing an AI program.
This program could successfully tell the difference between burned and unburned flint, even reporting on how the material got hot. The researchers, alongside scientists from the Hebrew University of Jerusalem and the University of Toronto, traveled to the Evron Quarry (an open-air archeological site in northern Israel) to test their program.
They found that 26 flint tools previously discovered at the Evron Quarry had been exposed to extremely high temperatures, some as high as 600 degrees Celsius. The findings support the “cooking hypothesis”, which is a widely accepted belief that harnessing fire was an important step in human evolution.