Build safe, and trustworthy AI at scale [OnDemand]
Build performant, safe, and trustworthy AI at scale [OnDemand]
Whether you buy AI models from external vendors or build them internally, the key technical challenges remain the same – expensive to obtain datasets, huge class imbalance, data shifts, data leakage or model degradation after deployment.
So, how do you know what is causing your AI models to fail, and how do you stop that from happening?
Discover all the answers and more by catching the replay of our live session with LatticeFlow.
3 key takeaways:
- Buy or build: Regardless if you buy or build, understanding your AI model failures and risks is critical.
- The necessity of identifying hidden blind spots: Preemptively identifying hidden model blind spots before deployment is becoming essential.
- Understand your data at scale: To help you make informed decisions.
Meet your expert host...
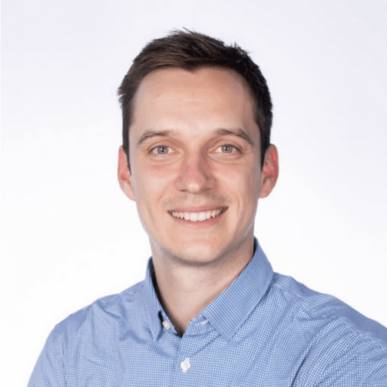
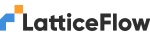