How to set up an efficient data flywheel for machine learning [OnDemand]
Setting up a fully automated machine learning pipeline is a must for everyone who wants to scale their ML product successfully.
But how are you closing the feedback loop from storage over training to deployment? It takes several steps to get there.
So, if you’re interested in:
→ Selecting data for model training in the best possible way.
→ Monitoring models in production and detecting drift.
→ Smart data mining from production serving data (edge or cloud).
→ Managing training and test datasets to prevent data leakage.
→ The benefits of a feedback loop.
Then we've got the session for you.
Catch the replay of our live session with the co-founder of Lightly AI as we delve into all the techniques you can use to automate your ML pipeline and scale your product.
Key takeaways
🔢 Selecting the best data for model training automatically
⚙️ Monitoring models in production and detecting drift
🔄 Benefits of a feedback loop
Meet our expert host
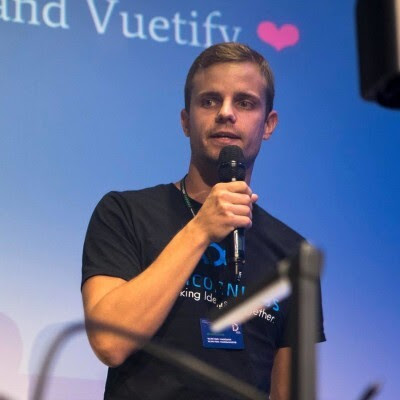