Another month has come and gone!
In this month’s edition of our news roundup series, we’ll cover a few topics in machine learning, deep learning, and computer vision, from healthcare to aerospace.
Read on to learn how:
- A record-breaking chip can transmit the entire internet's traffic per second
- Machine learning may help predict ALS progression
- A deep learning algorithm can detect cancer spread following surgery
- A deep learning tool could help with monkeypox, skin disease identification
- Computer vision takes flight for pilot awareness
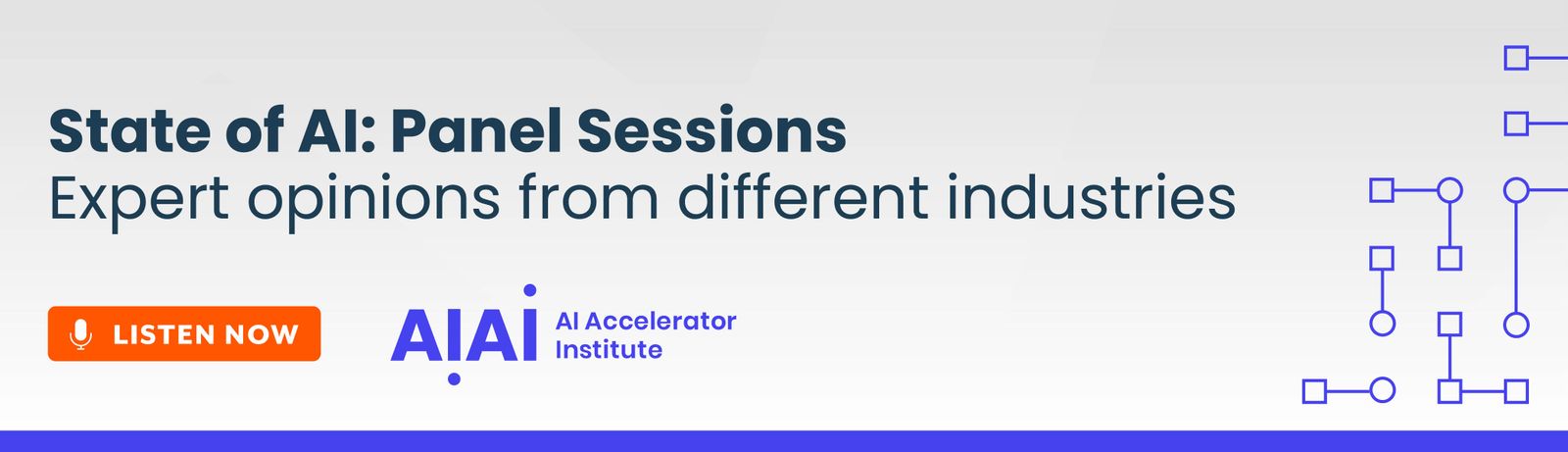
A record-breaking chip can transmit the entire internet's traffic per second
Researchers from the Technical University of Denmark (DTU) and the Chalmers University of Technology have now transmitted data at a rate of 1.84 petabits per second (Pbit/s) – almost double the global internet traffic per second – encoded in 223 wavelength channels down a 4.9-mile optical fiber containing 37 separate cores.
But how much is 1 petabit? That’s about a million gigabits, which is 20 times faster than ESnet6, which is the upgrade to the scientific network that NASA, for example, uses.
To set the new speed record, the team used a single light source and optical chip. An infrared laser is beamed into a chip, which is called a frequency comb, that then splits light into hundreds of different frequencies – or colors.
Data is encoded into the light by modulating the phase, amplitude, and polarization of each frequency, before recombining them into just one beam and transmitting it through an optical fiber.
Machine learning may help predict ALS progression
“Deep learning methods to predict amyotrophic lateral sclerosis disease progression”, a study published in Scientific Reports, highlights that deep machine learning models using neural networks might be able to help predict the course of amyotrophic lateral sclerosis (ALS).
Emphasizing how vital an early diagnosis can be, the models consistently showcased that a longer delay between ALS’s onset and the diagnosis can be a big predictor of worse long-term outcomes.
The Italian scientists created and tested three different types of neural network structures: recurrent, feed-forward, and convolutional, using information from over 250 different clinical and demographic variables.
Data from the Pooled Resource Open-Access ALS Clinical Trials (PRO-ACT) repository helped develop and test the models. The neural networks were trained to predict outcomes based on the ALS Functional Rating Scale (ALSFRS), which is a standardized measure of the disease’s progression.
The neural network algorithms were compared against the previously established machine learning ones, called Bayesian Additive Regression Trees (BART) and Random Forest Regressor (RF), showing smaller ranges of error but slightly less overall accuracy.
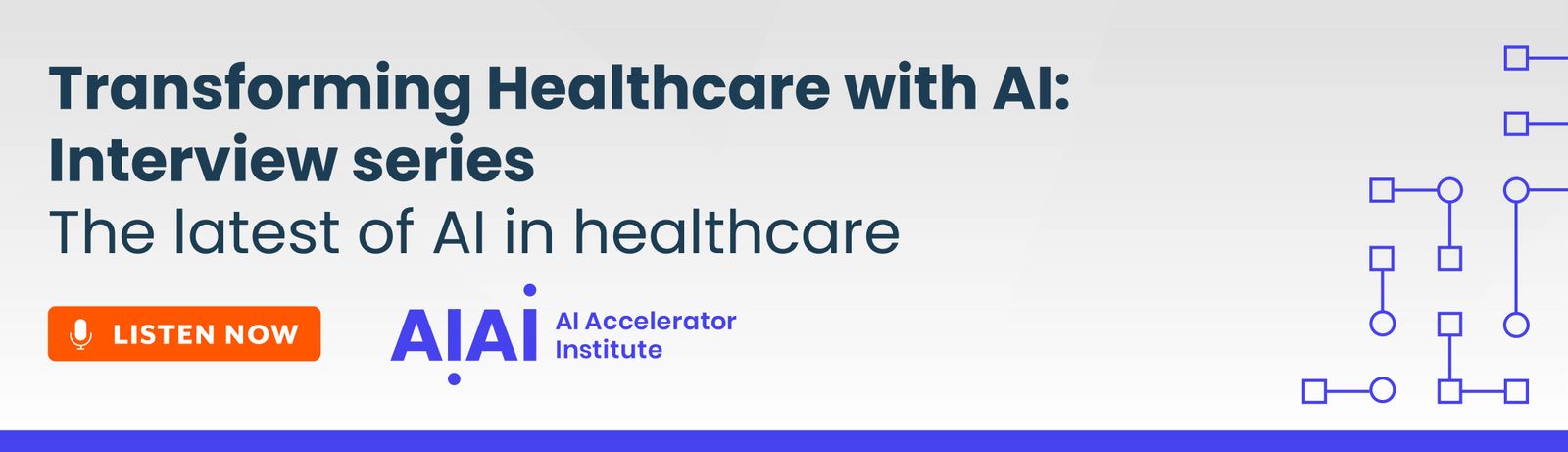
A deep learning algorithm can detect cancer spread following surgery
ECOG-ACRIN Cancer Research Group conducted a study in which it found that a deep learning algorithm that leverages standard computer tomography (CT) scan images can help assess head and neck cancer spread risk.
There’s a high degree of morbidity connected with standard neck and head cancer treatment, and researchers wanted to create an artificial intelligence tool that was able to predict the spread risk – allowing for accurate staging of the disease.
A neural network-based deep learning algorithm used standard CT images alongside other patient data from a previous trial, E3311. The number of lymph nodes, tumor size, and extranodal extension (ENE), for example, helped to determine the cancer stage.
The deep learning algorithm managed to accurately classify 85% of nodes as having ENE, a marked rise from 70% by radiologists.
A deep learning tool could help with monkeypox, skin disease identification
“Human Monkeypox Classification from Skin Lesion Images with Deep Pre-trained Network using Mobile Application”, a study published in the Journal of Medical Systems reported that a deep learning-based mobile application can accurately spot monkeypox infection with skin lesion images.
This smallpox-like disease causes chills, fever, lymph node swelling, chills, fatigue, painful skin eruptions, muscle aches, and respiratory symptoms, with the 2022 global outbreak declared a Public Health Emergency of International Concern (PHEIC).
The researchers’ aim was to develop a preliminary diagnostic tool to accurately identify monkeypox, so that infected individuals could seek a provider and isolate themselves faster. The result? An Android mobile application using artificial intelligence to analyze skin lesion images that are taken with the mobile device’s camera.
The team leveraged a deep convolutional network (CNN), using image pre-processing, transfer learning, and other methods to train the network.
The tool has average inference times of 197, 91, and 138 milliseconds, classifying images with a 91.11% accuracy.
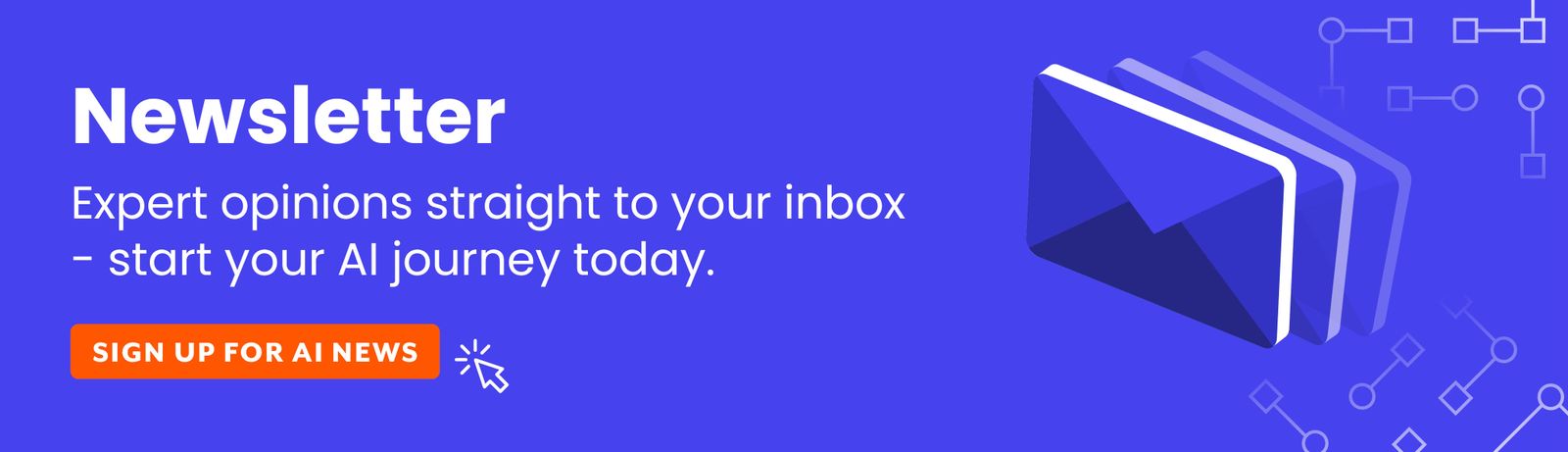
Computer vision takes flight for pilot awareness
The Swiss institute CSEM developed an eye-tracking and gesture recognition system for pilots. This computer vision system was built under the EU project Peggasus (Pilot Eye Gaze and Gesture tracking for Avionics Systems using Unobtrusive Solutions) and it was tested by ten pilots in a Lufthansa cockpit simulator.
The dashboard-mounted camera tracks pilots’ gazes and recognizes hand gestures in real-time, giving the pilots feedback on how to improve situational awareness when executing complicated tasks.
A deep neural network model was developed and incorporated into the final system to enable hand-gesture recognition. The system makes it easier for the flight crew to work aircraft controls, with the algorithm combining both analytical and data-driven approaches.
It operates in real-time at 60 frames per second and with minimum latency, achieving eye-tracking accuracy of better than 1.